Radio signal counterfeiting method for counterfeiting deep learning
A radio signal and deep learning technology, applied in neural learning methods, interference to communication, confidential communication, etc., can solve problems such as easy detection, large visual difference of fake signals, and inability to directly transfer signals, and reduce accuracy. Effect
- Summary
- Abstract
- Description
- Claims
- Application Information
AI Technical Summary
Problems solved by technology
Method used
Image
Examples
Embodiment 1
[0028] In electronic warfare, as the accuracy of signal classifiers based on deep learning continues to improve, the enemy's classification accuracy of radio signal modulation is getting higher and higher, and communication security is threatened. However, the existing data forgery methods against deep learning , which is mainly used against image classifiers in the field of computer vision. When used to forge signals, there will be a significant visual difference between the forged signal and the original radio signal. The enemy can identify the existence of the forged signal with the naked eye, reducing the deception of the forged signal properties, therefore, cannot be directly transferred to adversarial radio signal classifiers. From the perspective of eliminating the visual difference between the forged signal and the original radio signal, the present invention studies the radio signal forgery method for countering deep learning, proposes the visual limitation of signal f...
Embodiment 2
[0054]The overall technical scheme of the radio signal forgery method for combating deep learning is the same as that of embodiment 1, and the visual limitation of countering signal generation described in step (5) is applied to the differential evolution algorithm to obtain the signal-to-noise scheme set N', which is proposed by The visual restriction of counter signal generation, and the visual restriction is used in the differential evolution algorithm, by using the signal classifier M based on deep learning to evaluate the noise scheme, and finally generate the final population T' representing the signal-noise scheme set, see figure 2 , the specific implementation steps are:
[0055] (5a) Generate a population of offspring with visual constraints Applying the proposed visual constraints on adversarial signal generation to a differential evolution algorithm to generate offspring populations Generate a child population of the differential evolution algorithm for the pare...
Embodiment 3
[0071] The overall technical scheme of the radio signal forgery method for resisting deep learning is the same as embodiment 1-2, the evaluation noise scheme described in step (5b), is to adopt the signal classifier M based on deep learning, calculate T and The evaluation score of the middle noise scheme, the implementation steps include:
[0072] (5b1) Convert the interference scheme into a candidate countermeasure signal: the current parent population T and the child population The interference scheme in is transformed into a candidate countermeasure signal set X'=[x 1 ',...,x k ',...,x 800 '], where, 1≤k≤800, is the kth candidate adversarial signal, The conversion rules are:
[0073]
[0074]
[0075] (5b2) Obtain the evaluation score: input each candidate adversarial signal in the candidate counterfeit signal set to the signal classifier M based on deep learning to be adversarial, as the evaluation score of the corresponding interference scheme:
[0076] Sc...
PUM
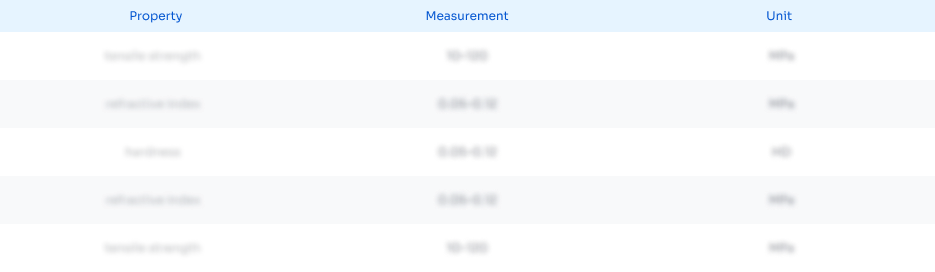
Abstract
Description
Claims
Application Information
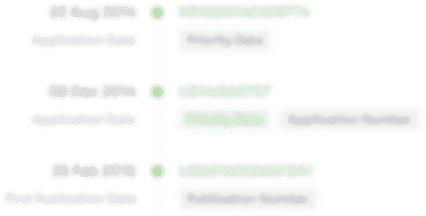
- R&D
- Intellectual Property
- Life Sciences
- Materials
- Tech Scout
- Unparalleled Data Quality
- Higher Quality Content
- 60% Fewer Hallucinations
Browse by: Latest US Patents, China's latest patents, Technical Efficacy Thesaurus, Application Domain, Technology Topic, Popular Technical Reports.
© 2025 PatSnap. All rights reserved.Legal|Privacy policy|Modern Slavery Act Transparency Statement|Sitemap|About US| Contact US: help@patsnap.com