Wind power plant short-term power prediction method based on feature selection and multi-level deep transfer learning
A technology of transfer learning and power prediction, applied in neural learning methods, predictions, instruments, etc., can solve problems such as the impact of network model prediction accuracy, poor complex information processing effect, and less accumulated wind power operation data, so as to improve the accuracy of prediction models, The effect of reducing size and reducing nesting problems
- Summary
- Abstract
- Description
- Claims
- Application Information
AI Technical Summary
Problems solved by technology
Method used
Image
Examples
Embodiment Construction
[0029] The following is a specific description in conjunction with the drawings in the embodiments of the present invention. The purpose of the present invention is to provide a short-term power prediction method for wind farms based on feature selection and multi-level deep transfer learning.
[0030] Such as figure 1 As shown, a wind farm short-term power prediction method based on feature selection and multi-level deep transfer learning includes the following steps:
[0031] S1: Collect wind farm data for data division and correlation analysis. The specific steps include:
[0032] S1.1: Collect wind farm time step length of 15 minutes and 600 consecutive days of data;
[0033] S1.2: Divide the collected data into two data sets, of which 10% of the data is used as the target wind farm data for transfer learning, and 90% of the data is used as the source wind farm data for transfer learning;
[0034] S1.3: Establish a prediction model based on a small amount of data of the ...
PUM
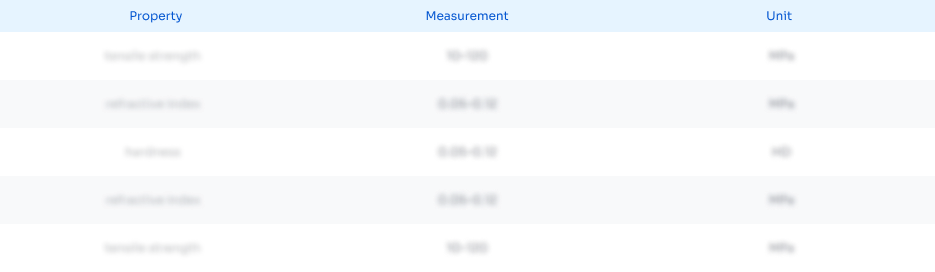
Abstract
Description
Claims
Application Information
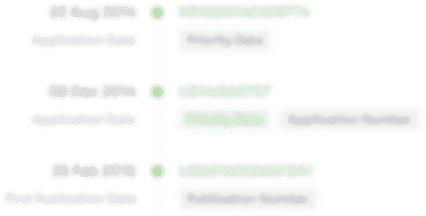
- R&D Engineer
- R&D Manager
- IP Professional
- Industry Leading Data Capabilities
- Powerful AI technology
- Patent DNA Extraction
Browse by: Latest US Patents, China's latest patents, Technical Efficacy Thesaurus, Application Domain, Technology Topic, Popular Technical Reports.
© 2024 PatSnap. All rights reserved.Legal|Privacy policy|Modern Slavery Act Transparency Statement|Sitemap|About US| Contact US: help@patsnap.com