Traffic organization scheme optimization method based on multi-agent reinforcement learning
A reinforcement learning, multi-agent technology, applied in neural learning methods, biological models, instruments, etc., can solve the problems of single experience processing, lack of communication information for multi-agent teamwork, easy congestion, etc., to improve efficiency and The effect of accuracy
- Summary
- Abstract
- Description
- Claims
- Application Information
AI Technical Summary
Problems solved by technology
Method used
Image
Examples
Embodiment 2
[0141] The present embodiment provides the example that utilizes the method in above-mentioned embodiment 1 to Mianyang CBD area prediction optimum traffic organization scheme:
[0142] data preparation:
[0143] Determine all vehicle trajectories and ODs in the Mianyang CBD area to be predicted, select the trajectory from 7:00 to 9:00 in the morning peak on September 2, 2019, the number of ODs in the morning peak is 78, and the minimum number of trajectories in an OD is 29, the maximum is 509, the length of the trajectory is also different, the longest is 30, and the average length is 15. After preparing to play OD data, train the maximum entropy inverse reinforcement learning traffic diversion model as the reward mechanism of the algorithm in this example.
[0144] Result analysis and display:
[0145] There are 210 road sections in the CBD area, of which 34 road sections are the key research objects. The criterion for judging the optimal plan is the difference between th...
PUM
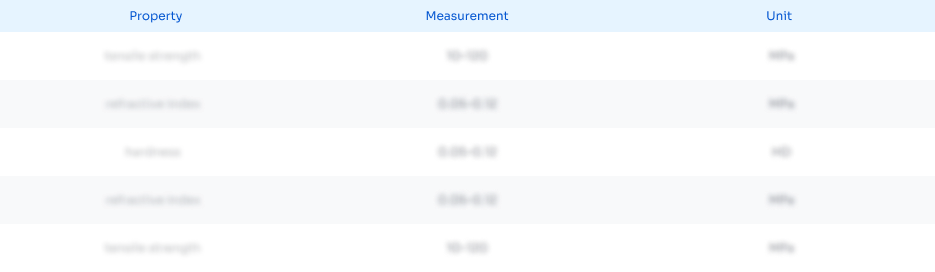
Abstract
Description
Claims
Application Information
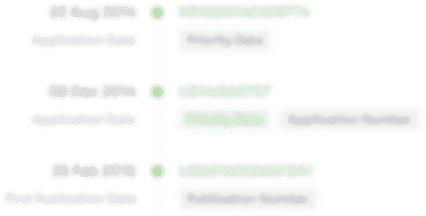
- R&D Engineer
- R&D Manager
- IP Professional
- Industry Leading Data Capabilities
- Powerful AI technology
- Patent DNA Extraction
Browse by: Latest US Patents, China's latest patents, Technical Efficacy Thesaurus, Application Domain, Technology Topic, Popular Technical Reports.
© 2024 PatSnap. All rights reserved.Legal|Privacy policy|Modern Slavery Act Transparency Statement|Sitemap|About US| Contact US: help@patsnap.com