Sparse graph coding-based hyperspectral remote sensing image eigen decomposition method and system
A hyperspectral remote sensing and sparse map technology, which is applied in the field of hyperspectral remote sensing image eigendecomposition method and system, can solve the problems of inability to effectively maintain the boundaries of ground objects and low precision, so as to maintain the boundaries of ground objects, improve accuracy, and avoid aliasing effect
- Summary
- Abstract
- Description
- Claims
- Application Information
AI Technical Summary
Problems solved by technology
Method used
Image
Examples
specific Embodiment approach 1
[0021] Specific implementation mode 1: This implementation mode is based on the hyperspectral remote sensing image eigendecomposition method based on sparse graph coding, and the specific process is as follows:
[0022] Step 1. Constructing a sparse graph coding dictionary for hyperspectral remote sensing images, including the following steps:
[0023] Step 11. Obtain hyperspectral remote sensing images:
[0024]
[0025] Among them, H=[H 1 ,H 2 ,...,H n ]∈R d×n , H is the hyperspectral remote sensing image, n is the total number of pixels on the hyperspectral remote sensing image, d represents the spectral dimension of the hyperspectral remote sensing image H, R d×n is a collection of matrices of size d×n on the real number field, ρ is the reflectivity component, 1 n is an n×1 all-one-column vector, is a 1×n full 1-row vector, 1 d is a full 1-column vector of d×1, β=[β 1 ,β 2 ,...,β n ] T is the column vector of the n×1 direction matrix, β T is the row vector ...
specific Embodiment approach 2
[0053]Specific Embodiment 2: The hyperspectral remote sensing image eigendecomposition system based on sparse graph coding is used to realize the hyperspectral remote sensing image eigendecomposition method based on sparse graph coding. The system includes: acquisition module, construction module, calculation module, decomposition module( image 3 );
[0054] The acquisition module is used to acquire hyperspectral remote sensing images;
[0055] The construction module is used to construct a sparse graph coding dictionary of a hyperspectral remote sensing image;
[0056] The calculation module is used to solve the similarity matrix of the sparse graph;
[0057] The decomposition module is used to decompose and obtain the reflectance components of the hyperspectral remote sensing image.
specific Embodiment approach 3
[0058] Specific embodiment three: the acquisition module is used to acquire hyperspectral remote sensing images, including the following steps:
[0059]
[0060] Among them, H=[H 1 ,H 2 ,...,H n ]∈R d×n , H is the hyperspectral remote sensing image, n is the total number of pixels on the hyperspectral remote sensing image, d represents the spectral dimension of the hyperspectral remote sensing image H, R d×n is a collection of matrices of size d×n on the real number field, ρ is the reflectivity component, 1 n is an n×1 all-one-column vector, is a 1×n full 1-row vector, 1 d is a full 1-column vector of d×1, β=[β 1 ,β 2 ,...,β n ] T is the column vector of the n×1 direction matrix, β T is the row vector of the n×1 direction matrix, E is the ambient light, E=[E(λ 1 ), E(λ 2 ),…,E(λ d )] T ∈ R d×1 , λ represents the wavelength of light.
[0061] Other steps are the same as in the second embodiment.
PUM
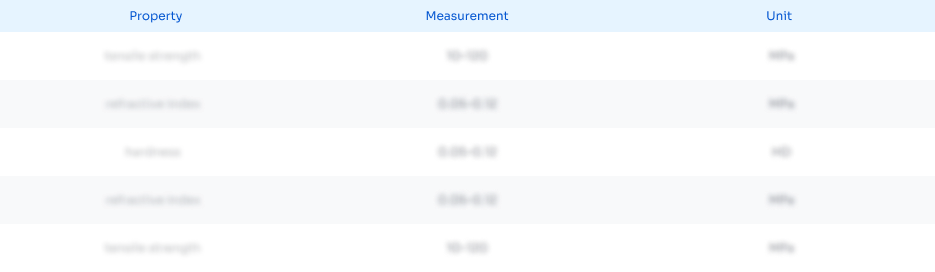
Abstract
Description
Claims
Application Information
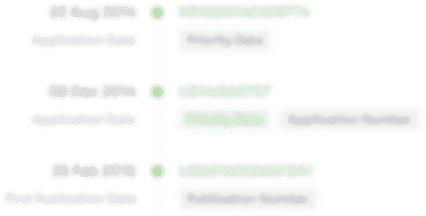
- R&D Engineer
- R&D Manager
- IP Professional
- Industry Leading Data Capabilities
- Powerful AI technology
- Patent DNA Extraction
Browse by: Latest US Patents, China's latest patents, Technical Efficacy Thesaurus, Application Domain, Technology Topic, Popular Technical Reports.
© 2024 PatSnap. All rights reserved.Legal|Privacy policy|Modern Slavery Act Transparency Statement|Sitemap|About US| Contact US: help@patsnap.com