An efficient robotic arm grasping deep reinforcement learning reward training method and system
A technology of reinforcement learning and training methods, applied in the field of machine learning, can solve problems such as incoordination and poor movement coherence, and achieve the effect of improving poor movement coherence and solving complex calculations
- Summary
- Abstract
- Description
- Claims
- Application Information
AI Technical Summary
Problems solved by technology
Method used
Image
Examples
Embodiment 1
[0041] In the step S1, after the depth camera identifies the end effector of the manipulator and the target object, it returns the coordinates of the end effector of the manipulator to the computer (x h ,y h ), depth d h , the coordinates of the target object (x o ,y o ), depth d o , and use the Euclidean distance to calculate the distance d between the end effector of the manipulator and the target object. The calculation formula is as follows:
[0042]
Embodiment 2
[0044] The step S2 initializes the moving distance reward r of the end effector of the robotic arm relative to the target object 1 , step reward r 2 , the sum of the rotation angles of the servos for each degree of freedom of the manipulator is rewarded r 3 and whether to grab a successful reward r 4, so that the above values are all 0, then the total number of rewards R is:
[0045] R=αr 1 +βr 2 +γr 3 +δr 4 (2).
Embodiment 3
[0047] The reward method calculated in the state S in the step S5 is:
[0048] S51: Calculate the moving distance △d=d'-d of the end effector of the robotic arm relative to the target object, and the moving distance is rewarded r 1 =-Δd;
[0049] S52: number of steps s=s+1, reward r for number of steps 2 =-s;
[0050] S53: Calculate the sum of the rotation angles of the servos for each degree of freedom of the manipulator as but
PUM
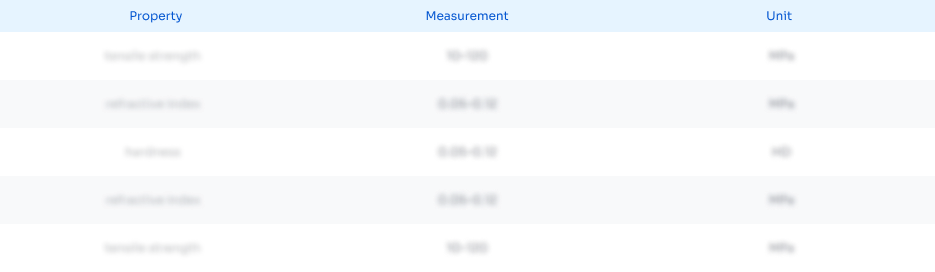
Abstract
Description
Claims
Application Information
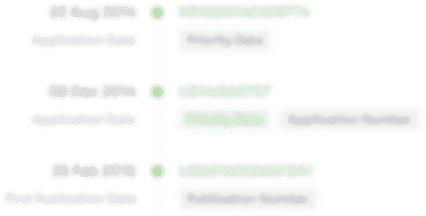
- R&D
- Intellectual Property
- Life Sciences
- Materials
- Tech Scout
- Unparalleled Data Quality
- Higher Quality Content
- 60% Fewer Hallucinations
Browse by: Latest US Patents, China's latest patents, Technical Efficacy Thesaurus, Application Domain, Technology Topic, Popular Technical Reports.
© 2025 PatSnap. All rights reserved.Legal|Privacy policy|Modern Slavery Act Transparency Statement|Sitemap|About US| Contact US: help@patsnap.com