Bearing fault diagnosis method and system based on sparse auto-encoder and Softmax
A sparse autoencoder and fault diagnosis technology, which is applied in the testing of machine/structural components, instruments, and mechanical components, etc. It can solve the problems of mining fault information, ignoring internal structure information, etc., and achieves high classification accuracy and structure. Simple and accurate results
- Summary
- Abstract
- Description
- Claims
- Application Information
AI Technical Summary
Problems solved by technology
Method used
Image
Examples
Embodiment Construction
[0045] The present invention will be further described in detail below in conjunction with the accompanying drawings, which are explanations rather than limitations of the present invention.
[0046] refer to Figure 1-3 , a bearing fault diagnosis method combining local sparse autoencoder and Softmax, including the following steps:
[0047] S1. Collect the time-domain vibration signals of rolling bearings in different operating states, and construct an unlabeled training set and a labeled training set according to the collected time-domain vibration signals.
[0048] Construct an unlabeled training set containing D samples and a labeled training set with F samples
[0049] where x d is the P-dimensional vibration signal collected in the training set T1; a f is the P-dimensional vibration signal collected in the training set T2, is the state label corresponding to the P-dimensional vibration signal, and C is the number of fault types of the bearing.
[0050] Specific...
PUM
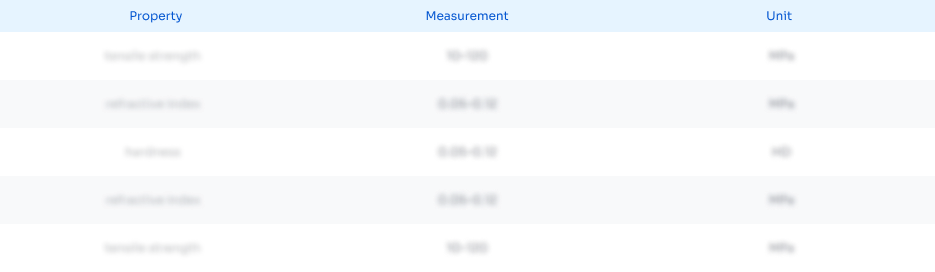
Abstract
Description
Claims
Application Information
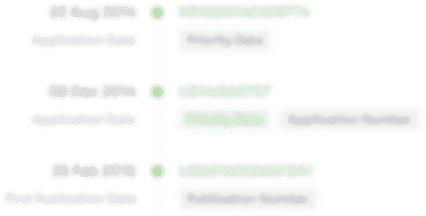
- R&D
- Intellectual Property
- Life Sciences
- Materials
- Tech Scout
- Unparalleled Data Quality
- Higher Quality Content
- 60% Fewer Hallucinations
Browse by: Latest US Patents, China's latest patents, Technical Efficacy Thesaurus, Application Domain, Technology Topic, Popular Technical Reports.
© 2025 PatSnap. All rights reserved.Legal|Privacy policy|Modern Slavery Act Transparency Statement|Sitemap|About US| Contact US: help@patsnap.com