Hail recognition method under multi-dimensional radar data based on semi-supervised learning
A semi-supervised learning and radar data technology, which is applied in the interdisciplinary field of computer artificial intelligence and meteorology, can solve the problems that the recognition effect needs to be improved, and achieve the effect of automatically optimizing the training model, improving the accuracy rate, and reducing the false alarm rate
- Summary
- Abstract
- Description
- Claims
- Application Information
AI Technical Summary
Problems solved by technology
Method used
Image
Examples
Embodiment 1
[0040] This embodiment discloses a hail recognition method based on semi-supervised learning under multi-dimensional radar data, comprising the following steps:
[0041] S1: Obtain the labeled sample set, extract the supervised sample set from the labeled sample set by random sampling, and divide the labeled sample set into the rainstorm sample training set and the hail sample training set according to the rainstorm and hail labels, and obtain the unlabeled data set. Divided into q first samples;
[0042] In this embodiment, the labeled sample set includes radar basic reflectivity images and Doppler weather radar series data;
[0043] Specifically, read in m labeled sample sets:
[0044]
[0045] Randomly selected from the labeled sample set samples, named Used to supervise the training process. Supervised sample set :
[0046]
[0047] According to the labeled sample labels, it is divided into rainstorm sample training set training set with hail samples :
...
Embodiment 2
[0088] Based on the method in Embodiment 1, a specific implementation is proposed in this embodiment to train and test the method in Embodiment 1:
[0089] In this embodiment, 104 initial hail training data and 103 hail test data are selected, 104 are randomly selected from 207 hail live data as training data, and the other 103 are used as test data; 1098 rainstorm training data are selected simultaneously, 1098 1098 training data and another 1098 are randomly divided from 2196 rainstorm real data as test data. The unknown sample data can be: The network collects the time when the hailstorm occurred in Chongqing in 2019, and analyzes the combined reflectance file near the time point, and obtains a total of 20,850 sample data, which is used as an unlabeled data set for training the recognition model;
[0090] The 20850 unknown sample data were randomly divided into 1000 groups, and then added to the training according to the method in Example 1. The weight values used in the ...
PUM
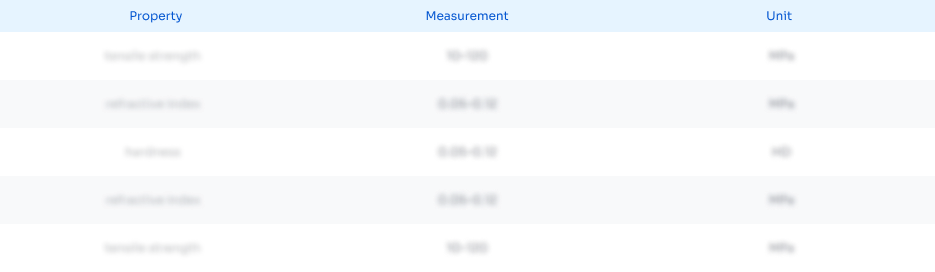
Abstract
Description
Claims
Application Information
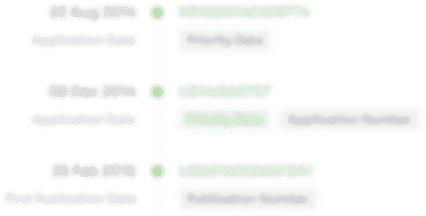
- R&D
- Intellectual Property
- Life Sciences
- Materials
- Tech Scout
- Unparalleled Data Quality
- Higher Quality Content
- 60% Fewer Hallucinations
Browse by: Latest US Patents, China's latest patents, Technical Efficacy Thesaurus, Application Domain, Technology Topic, Popular Technical Reports.
© 2025 PatSnap. All rights reserved.Legal|Privacy policy|Modern Slavery Act Transparency Statement|Sitemap|About US| Contact US: help@patsnap.com