An image blind super-resolution method and system
A technology for super-resolution and low-resolution images, applied in the field of image blind super-resolution methods and systems, can solve problems such as poor estimation accuracy, long running time, and complex calculations, and reduce difficulty and running time, convolution Ease of operation and improved estimation accuracy
- Summary
- Abstract
- Description
- Claims
- Application Information
AI Technical Summary
Problems solved by technology
Method used
Image
Examples
Embodiment 1
[0043] see figure 1 , this embodiment provides an image blind super-resolution method, the method includes the following steps:
[0044] Step 1: Obtain the trained blur kernel generation network and the spectrogram of the low-resolution image.
[0045] Step 2: Input the spectrogram into the trained blur kernel generation network to obtain a blur kernel corresponding to the low-resolution image.
[0046] Step 3: Determine the degradation feature map corresponding to the low-resolution image according to the blur kernel corresponding to the low-resolution image.
[0047] Step 4: splicing the low-resolution image and its corresponding degraded feature map to obtain a spliced map.
[0048] Step 5: Input the mosaic image into a trained convolutional neural network to obtain a high-resolution image.
[0049] In an example, before step 1, a step of determining the frequency domain map of the low-resolution image may also be included, specifically, Fourier transform may be used t...
Embodiment 2
[0086] see Figure 5 , this embodiment provides an image blind super-resolution system, the system includes:
[0087] an acquisition module 501, configured to acquire the trained fuzzy kernel generation network and the spectrogram of the low-resolution image;
[0088] A blur kernel determination module 502, configured to input the spectrogram into the trained blur kernel generation network to obtain a blur kernel corresponding to the low-resolution image;
[0089] A degradation feature map determining module 503, configured to determine a degradation feature map corresponding to the low-resolution image according to the blur kernel corresponding to the low-resolution image;
[0090] The image stitching module 504 is used for stitching the low-resolution image and its corresponding degradation feature map to obtain a stitching map;
[0091] The high-resolution image determination module 505 is configured to input the mosaic image into the trained dense convolutional neural ne...
PUM
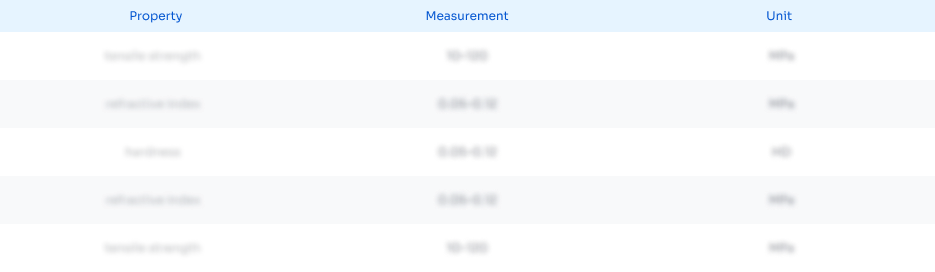
Abstract
Description
Claims
Application Information
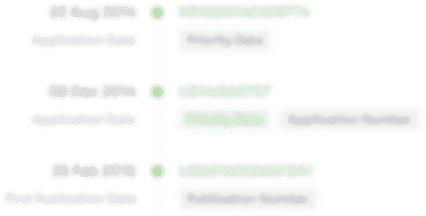
- R&D Engineer
- R&D Manager
- IP Professional
- Industry Leading Data Capabilities
- Powerful AI technology
- Patent DNA Extraction
Browse by: Latest US Patents, China's latest patents, Technical Efficacy Thesaurus, Application Domain, Technology Topic, Popular Technical Reports.
© 2024 PatSnap. All rights reserved.Legal|Privacy policy|Modern Slavery Act Transparency Statement|Sitemap|About US| Contact US: help@patsnap.com