Online extreme learning machine target identification method and system based on consistent regularization
An extreme learning machine and target recognition technology, which is applied in the field of online extreme learning machine target recognition method and system, can solve the problems of unsatisfactory effect performance, overfitting, and performance degradation of target recognition technology, and achieve good reliability. Scalability, improved robustness, and low computational cost
- Summary
- Abstract
- Description
- Claims
- Application Information
AI Technical Summary
Problems solved by technology
Method used
Image
Examples
Embodiment Construction
[0041] The present invention will be further described below in conjunction with the accompanying drawings and specific preferred embodiments, but the protection scope of the present invention is not limited thereby.
[0042] Such as figure 1 and figure 2 As shown, the online ELM target recognition method based on consistent regularization in this embodiment includes the following steps of learning and training a classifier based on a single hidden layer feedforward neural network:
[0043] S1. Acquire training images, perform feature extraction on the training images, obtain corresponding image feature sets, and randomly divide the image feature sets into multiple feature subsets;
[0044] S2. Generate corresponding neighbor feature samples for the divided feature subsets;
[0045] S3. Introduce the consistent regularization constraint into the online extreme learning objective optimization function, randomly generate the hidden layer node parameters (such as weight, bias...
PUM
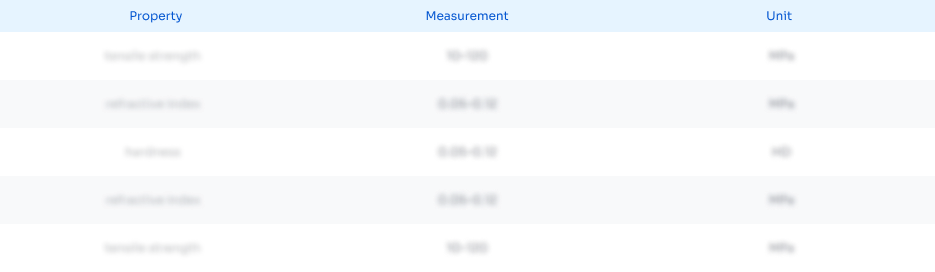
Abstract
Description
Claims
Application Information
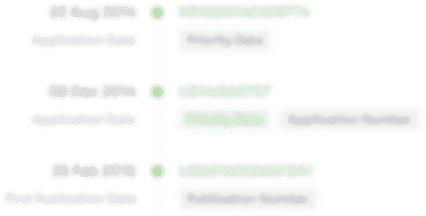
- R&D Engineer
- R&D Manager
- IP Professional
- Industry Leading Data Capabilities
- Powerful AI technology
- Patent DNA Extraction
Browse by: Latest US Patents, China's latest patents, Technical Efficacy Thesaurus, Application Domain, Technology Topic.
© 2024 PatSnap. All rights reserved.Legal|Privacy policy|Modern Slavery Act Transparency Statement|Sitemap