Lightweight unsupervised anomaly detection method based on multivariate time series data analysis
A technology for time series data and anomaly detection, applied in neural learning methods, instruments, biological neural network models, etc., can solve the problems of reduced practicability and stability, difficult to train, difficult to use, etc., to improve practicability and reduce model scale. , the effect of improving usability and stability
- Summary
- Abstract
- Description
- Claims
- Application Information
AI Technical Summary
Problems solved by technology
Method used
Image
Examples
Embodiment Construction
[0027] Below in conjunction with accompanying drawing and specific implementation steps, the present invention has been further described:
[0028] A lightweight unsupervised anomaly detection method based on multivariate time-series data analysis, comprising the following steps:
[0029] Step 1: Data preprocessing and segmentation. According to the set window parameters, the data is processed into a size corresponding to the window size to meet the requirements of the detection model for input data; the processed data is divided into training data and test data.
[0030] Such as figure 1 Shown, have shown the overall structure of the present invention. The data processing and segmentation part is at the entrance of the structure of the present invention, and is responsible for preliminary processing of the original data to form the data structure required by the detection model. It is worth noting that we count the window size as T+1, the test data x at time t t depends on...
PUM
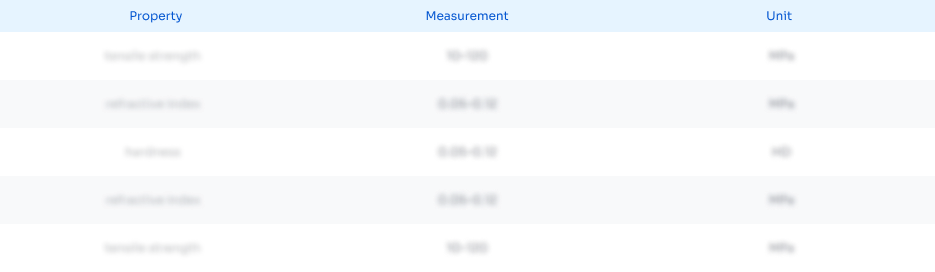
Abstract
Description
Claims
Application Information
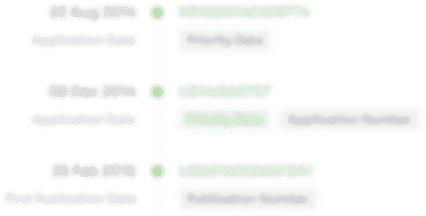
- R&D
- Intellectual Property
- Life Sciences
- Materials
- Tech Scout
- Unparalleled Data Quality
- Higher Quality Content
- 60% Fewer Hallucinations
Browse by: Latest US Patents, China's latest patents, Technical Efficacy Thesaurus, Application Domain, Technology Topic, Popular Technical Reports.
© 2025 PatSnap. All rights reserved.Legal|Privacy policy|Modern Slavery Act Transparency Statement|Sitemap|About US| Contact US: help@patsnap.com