Traffic speed prediction method based on time sequence diagram neural network
A neural network and speed prediction technology, which is applied in biological neural network models, traffic flow detection, traffic control systems of road vehicles, etc., can solve problems such as traffic congestion, traffic flow and traffic speed decline, and achieve ease of error transmission and improvement Learning ability, the effect of improving learning ability
- Summary
- Abstract
- Description
- Claims
- Application Information
AI Technical Summary
Problems solved by technology
Method used
Image
Examples
Embodiment
[0054]In this embodiment, the method of the present invention adopts the encoding and decoding framework to model the spatio-temporal features in traffic speed prediction, and the input layer of the encoding end performs feature transformation on the original node features; in the spatial feature fusion layer, it is mainly for each node in the network Model the spatial feature information of the node, use the network representation learning algorithm and the graph convolutional neural network to capture the spatial feature of the node, and then fuse it with the original input feature of the node; The temporal feature information of the encoding end and the hidden state of the input to the next moment are obtained; the input layer of the decoding end passes through a fully connected layer to convert the original features of the nodes in the training stage to a high-dimensional space; the features obtained by the input layer, attention The force result and the hidden state of the...
PUM
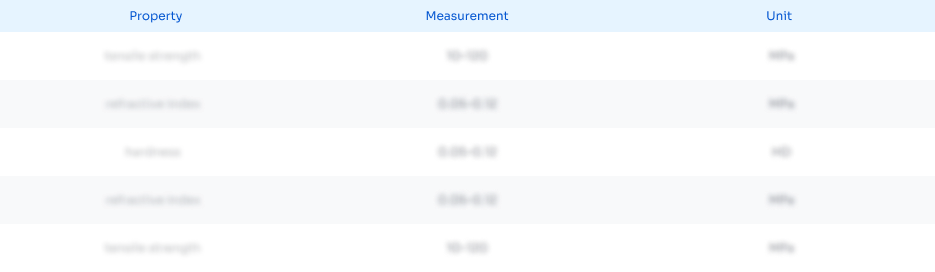
Abstract
Description
Claims
Application Information
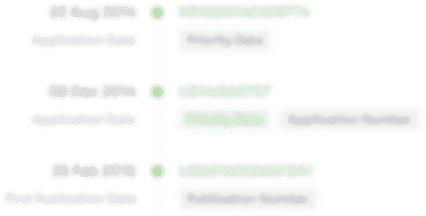
- R&D Engineer
- R&D Manager
- IP Professional
- Industry Leading Data Capabilities
- Powerful AI technology
- Patent DNA Extraction
Browse by: Latest US Patents, China's latest patents, Technical Efficacy Thesaurus, Application Domain, Technology Topic, Popular Technical Reports.
© 2024 PatSnap. All rights reserved.Legal|Privacy policy|Modern Slavery Act Transparency Statement|Sitemap|About US| Contact US: help@patsnap.com