Stock rise and fall prediction method based on multi-task self-supervised learning
A technology of supervised learning and prediction method, which is applied in the field of stock ups and downs prediction based on multi-task self-supervised learning, which can solve the problem that artificial features cannot make full use of stock signals, and achieve high accuracy.
- Summary
- Abstract
- Description
- Claims
- Application Information
AI Technical Summary
Problems solved by technology
Method used
Image
Examples
Embodiment 1
[0138] The stock market trend prediction method for learning stock sequence embeddings in self-supervised multi-task is carried out as follows:
[0139] S1: Collect stock data with daily frequency, including 6-dimensional data of highest price, lowest price, opening price, closing price, transaction volume, and transaction amount, and perform preprocessing such as de-extreme value and standardization on the collected data, and divide them into Training set, verification set and test set, the stock data from January 1, 2018 to December 31, 2019 is used as the training set, and 10% of the last time is divided from the training set as the verification set, and January 2020 is used The data from September 1st to September 30th, 2020 is used as the test set.
[0140] S2: The anchor-sample sequence is obtained by sampling in the data set. Taking Shanghai Pudong Development Bank as an example with the stock code 600000, when k=5, at time t=January 11, 2019, take the last 5 trading da...
PUM
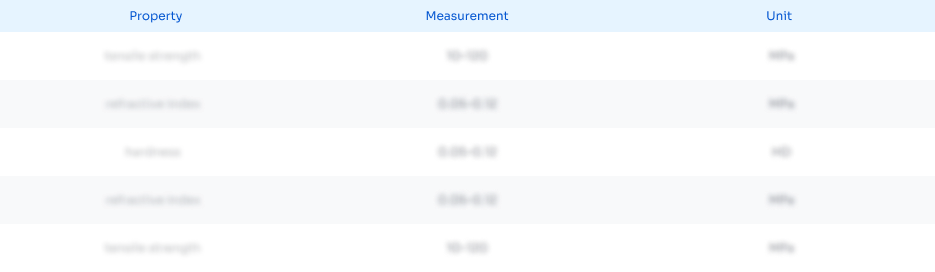
Abstract
Description
Claims
Application Information
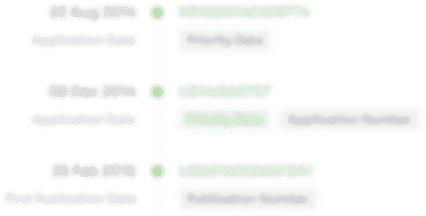
- R&D Engineer
- R&D Manager
- IP Professional
- Industry Leading Data Capabilities
- Powerful AI technology
- Patent DNA Extraction
Browse by: Latest US Patents, China's latest patents, Technical Efficacy Thesaurus, Application Domain, Technology Topic.
© 2024 PatSnap. All rights reserved.Legal|Privacy policy|Modern Slavery Act Transparency Statement|Sitemap