Vehicle re-identification method and system based on double attention mechanisms
An attention and re-identification technology, applied in the field of vehicle re-identification, can solve the problems of data overfitting, increase computing resources, reduce practicability, etc., and achieve fast convergence, robust fine-grained features, and accelerated model training. Effect
- Summary
- Abstract
- Description
- Claims
- Application Information
AI Technical Summary
Problems solved by technology
Method used
Image
Examples
Embodiment 1
[0081] The data in this embodiment adopts the Veri-776 data set. The images in the data set come from urban surveillance videos. 20 cameras cover an area of one square kilometer, and the monitoring time is 24 hours. There are 776 vehicles in this dataset, and the total number of images is 49357, of which the number of training images is 37778, the number of query images is 1678, and the number of test images is 11579. The detailed information of the data set is shown in Table 1 below. This embodiment is mainly implemented based on the framework Pytorch1.5.1. The graphics card used in the experiment is GEFORCERTX 2080Ti, the system is Ubuntu 20.04, the CUDA version is 10.2, and the cudnn version is 8.0.1.
[0082] Table 1 Detailed information table of vehicle re-identification dataset
[0083]
[0084] Such as figure 1 As shown, the present embodiment provides a vehicle re-identification method based on a dual attention mechanism, comprising the following steps:
[0085]...
Embodiment 2
[0121] This embodiment provides a vehicle re-identification system based on a dual attention mechanism, including: a convolutional neural network building block, a channel attention mechanism component building block, a granular attention mechanism component building block, a batch image building block, real-time data Enhancement modules, loss function building blocks, convolutional neural network training modules, and reranking modules;
[0122] The convolutional neural network building block is used to build a convolutional neural network, and the convolutional neural network is used for vehicle feature extraction; the channel attention component building block is used to build a channel attention component, and assign different weights to different channels; the granular attention component building block Used to build granular attention components, focusing on the most suitable granular representation of features; the batch image building module is used to randomly select m...
PUM
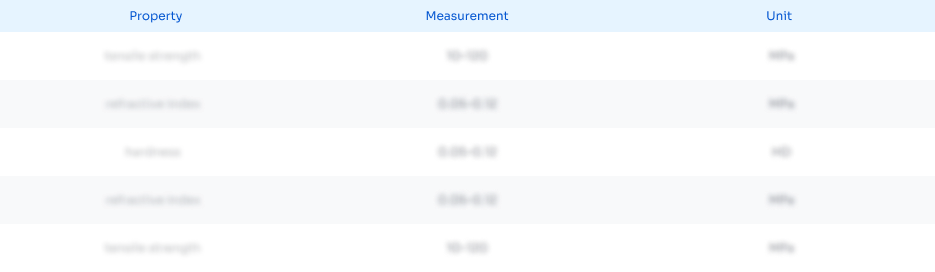
Abstract
Description
Claims
Application Information
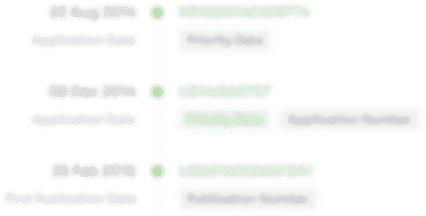
- R&D Engineer
- R&D Manager
- IP Professional
- Industry Leading Data Capabilities
- Powerful AI technology
- Patent DNA Extraction
Browse by: Latest US Patents, China's latest patents, Technical Efficacy Thesaurus, Application Domain, Technology Topic, Popular Technical Reports.
© 2024 PatSnap. All rights reserved.Legal|Privacy policy|Modern Slavery Act Transparency Statement|Sitemap|About US| Contact US: help@patsnap.com