Photovoltaic power generation power prediction method based on long short-term memory neural network
A photovoltaic power generation, long-term and short-term memory technology, applied in neural learning methods, biological neural network models, prediction and other directions, can solve the problems of low prediction accuracy, poor generalization and robustness, and achieve the effect of improving prediction accuracy
- Summary
- Abstract
- Description
- Claims
- Application Information
AI Technical Summary
Problems solved by technology
Method used
Image
Examples
Embodiment Construction
[0042] The present invention will be further explained below with reference to the embodiments and accompanying drawings, but it is not intended to limit the scope of the present application once.
[0043] The present invention includes the following steps:
[0044] Step 1: Obtain the historical data of photovoltaic power in a certain area for a period of time, and use the obtained data as a data set;
[0045] Step 2: Process the data in the data set in Step 1, remove abnormal data, divide the remaining data into training set and test set by K-fold cross-validation method, and perform normalization processing;
[0046] Step 3: After normalization, determine the number of neurons in the input layer, hidden layer and output layer of the long short-term memory neural network, and determine the number of quantiles in the photovoltaic power generation power prediction model;
[0047] The photovoltaic power generation power prediction model of the invention combines the long-short-...
PUM
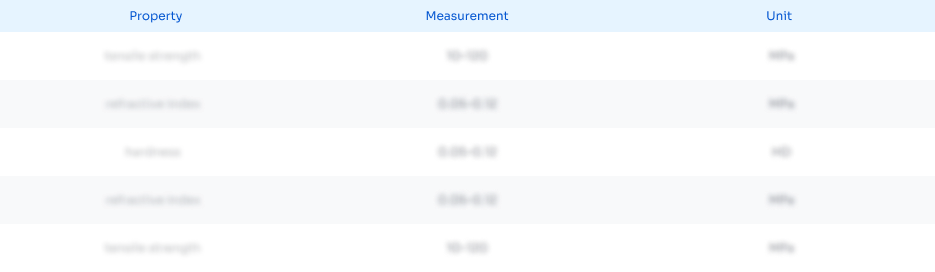
Abstract
Description
Claims
Application Information
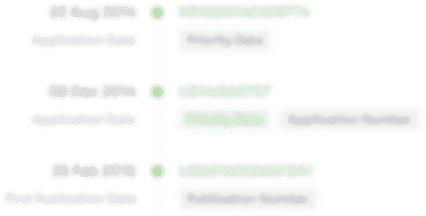
- R&D
- Intellectual Property
- Life Sciences
- Materials
- Tech Scout
- Unparalleled Data Quality
- Higher Quality Content
- 60% Fewer Hallucinations
Browse by: Latest US Patents, China's latest patents, Technical Efficacy Thesaurus, Application Domain, Technology Topic, Popular Technical Reports.
© 2025 PatSnap. All rights reserved.Legal|Privacy policy|Modern Slavery Act Transparency Statement|Sitemap|About US| Contact US: help@patsnap.com