Logistics risk assessment model method and device based on dynamic Bayesian network, equipment and medium
A dynamic Bayesian and Bayesian network technology, applied in the field of logistics intelligence, can solve problems such as the inability to realize timely discovery and correct evaluation of logistics, and achieve the effect of improving the evaluation effect and realizing the effect of risk management and control.
- Summary
- Abstract
- Description
- Claims
- Application Information
AI Technical Summary
Problems solved by technology
Method used
Image
Examples
Embodiment 1
[0040] The embodiment of the present application provides a logistics risk assessment model method based on a dynamic Bayesian network, such as figure 1 shown, including:
[0041] Step S100, determine a number of logistics links, and determine candidate risk factors associated with each logistics link to Form a set of candidate risk influencing factors.
[0042] Optionally, according to the possible sources of risk, the logistics process is divided into seven links, including product packaging, distribution processing, logistics warehousing, loading and unloading, product transportation, product distribution and logistics information. Except for logistics information accidents, the other six links occur sequentially over time.
[0043] The above seven logistics links all include certain risk factors associated with them. For example, the product packaging link includes packaging specifications, packaging environment, packaging operations, etc., and logistics warehousing ...
Embodiment 2
[0093] Figure 4 A schematic structural diagram of the logistics risk assessment module 10 based on the dynamic Bayesian network provided in this embodiment.
[0094] The flow risk assessment module 10 includes:
[0095] The candidate risk influencing factor determining module 11 is configured to determine several logistics links, and determine candidate risk influencing factors associated with each logistics link to form a set of candidate risk influencing factors.
[0096] The formal risk influencing factor selection module 12 is configured to select a number of candidate risk influencing factors from the set of candidate risk influencing factors as formal risk influencing factors.
[0097] A priori probability determination module 13, configured to determine the initial state category of each of the formal risk influencing factors and the prior probability value corresponding to each of the initial state categories;
[0098] The static Bayesian network construction module...
Embodiment 3
[0104] Figure 5 A schematic structural diagram of the electronic device 20 provided in the embodiment of the present application, such as Figure 5 As shown, the electronic device 20 includes a processor 21 and a memory 23 , and the processor 21 and the memory 23 are connected, such as through a bus 22 .
[0105] The processor 21 may be a CPU, a general purpose processor, DSP, ASIC, FPGA or other programmable devices, transistor logic devices, hardware components or any other combination. It can implement or execute the various illustrative logical blocks, modules and circuits described in connection with the present disclosure. The processor 21 may also be a combination of computing functions, for example, a combination of one or more microprocessors, a combination of a DSP and a microprocessor, and the like.
[0106] Bus 22 may include a path for communicating information between the components described above. The bus 22 may be a PCI bus or an EISA bus or the like. The...
PUM
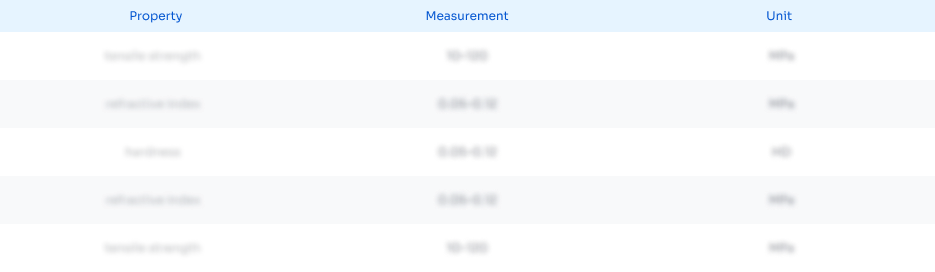
Abstract
Description
Claims
Application Information
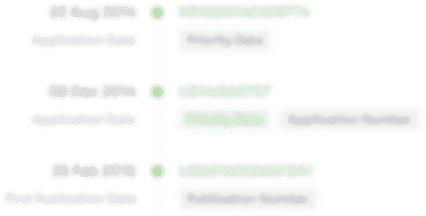
- R&D
- Intellectual Property
- Life Sciences
- Materials
- Tech Scout
- Unparalleled Data Quality
- Higher Quality Content
- 60% Fewer Hallucinations
Browse by: Latest US Patents, China's latest patents, Technical Efficacy Thesaurus, Application Domain, Technology Topic, Popular Technical Reports.
© 2025 PatSnap. All rights reserved.Legal|Privacy policy|Modern Slavery Act Transparency Statement|Sitemap|About US| Contact US: help@patsnap.com