Air quality prediction method based on seasonal recurrent neural network
A cyclic neural network and air quality technology, applied in the field of electronic information, can solve the problems of limited prediction accuracy and interpretability, not considering the periodicity of air quality monitoring time series, seasonal characteristics, etc., to achieve the effect of improving prediction accuracy
- Summary
- Abstract
- Description
- Claims
- Application Information
AI Technical Summary
Problems solved by technology
Method used
Image
Examples
Embodiment Construction
[0016] In order to make the purpose, technical solution and advantages of the present invention clearer, the embodiments of the present invention will be further described below in conjunction with the accompanying drawings.
[0017] Please refer to figure 1 , the present invention provides figure 1 It is a kind of air quality prediction method based on seasonal recurrent neural network of the present invention; Specifically comprise the following steps:
[0018] S1. Record long-sequence air quality monitoring site data; such as temperature, wind speed, PM2.5 monitoring parameter information, monitoring location information, monitoring time information, and site ID, etc., and establish a relational database, wherein the monitoring location is represented by latitude and longitude coordinates; The monitoring time information is sampling time information;
[0019] S2. Preprocessing the data of long-term air quality monitoring stations, including imputation of missing values ...
PUM
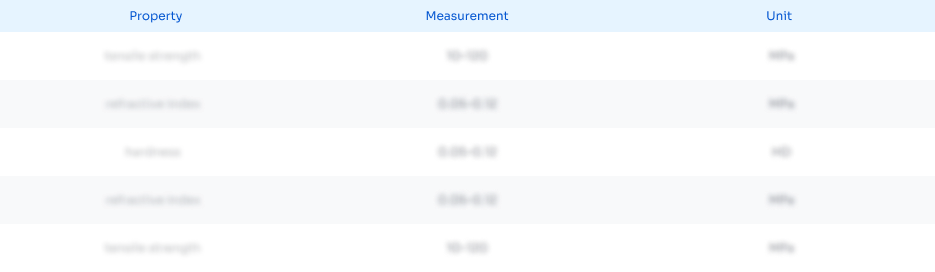
Abstract
Description
Claims
Application Information
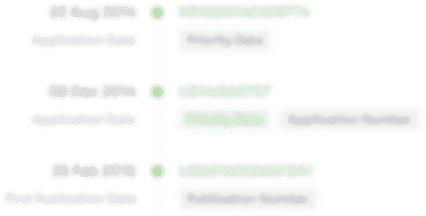
- R&D Engineer
- R&D Manager
- IP Professional
- Industry Leading Data Capabilities
- Powerful AI technology
- Patent DNA Extraction
Browse by: Latest US Patents, China's latest patents, Technical Efficacy Thesaurus, Application Domain, Technology Topic, Popular Technical Reports.
© 2024 PatSnap. All rights reserved.Legal|Privacy policy|Modern Slavery Act Transparency Statement|Sitemap|About US| Contact US: help@patsnap.com