Neural component searching method for generating image super-resolution network
A technology for image generation and search methods, applied in the field of neural component search, can solve problems such as network structure and loss function differences, large search space, long search time, etc., to speed up search time, expand flexibility, and reduce prior knowledge Introduced effects
- Summary
- Abstract
- Description
- Claims
- Application Information
AI Technical Summary
Problems solved by technology
Method used
Image
Examples
Embodiment Construction
[0059] The present invention will be further explained below in conjunction with the accompanying drawings and specific embodiments. It should be understood that the following specific embodiments are only used to illustrate the present invention and are not intended to limit the scope of the present invention.
[0060] refer to figure 1 , the specific implementation steps of the neural component search method for generating an image super-resolution network proposed by the present invention are as follows.
[0061] Step S1: Design the search space The search space is a hypergraph to be searched, including search possible network nodes, connection paths, loss functions 144-147, and color space. The order of different network nodes has a great influence on the results. For example, the order of convolutional layer, activation layer, and normalization layer is usually fixed, and too free search space will greatly increase the difficulty of neural component search methods. , so ...
PUM
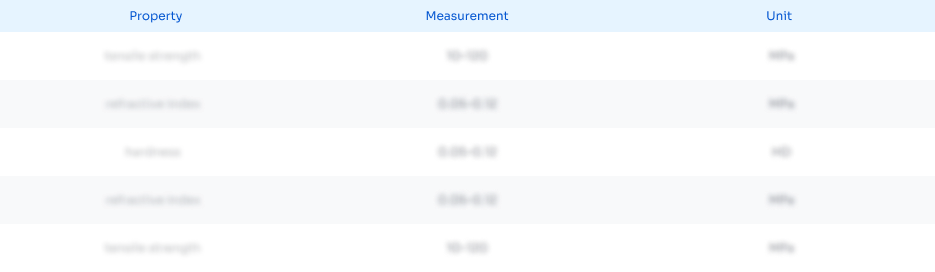
Abstract
Description
Claims
Application Information
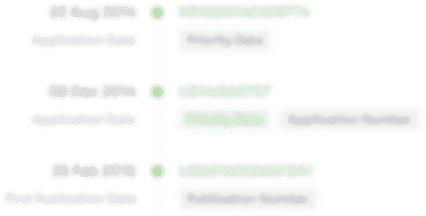
- Generate Ideas
- Intellectual Property
- Life Sciences
- Materials
- Tech Scout
- Unparalleled Data Quality
- Higher Quality Content
- 60% Fewer Hallucinations
Browse by: Latest US Patents, China's latest patents, Technical Efficacy Thesaurus, Application Domain, Technology Topic, Popular Technical Reports.
© 2025 PatSnap. All rights reserved.Legal|Privacy policy|Modern Slavery Act Transparency Statement|Sitemap|About US| Contact US: help@patsnap.com