Long-term network traffic forecasting method based on deep learning
A network traffic, deep learning technology, applied in the field of wireless communication, can solve the problems of long-term prediction accuracy decline, error accumulation, poor effect, etc., to achieve the effect of being conducive to reasonable distribution, improving accuracy, and reducing data inconsistency
- Summary
- Abstract
- Description
- Claims
- Application Information
AI Technical Summary
Problems solved by technology
Method used
Image
Examples
Embodiment Construction
[0062] In order to describe the technical solution disclosed in the present invention in detail, further elaboration will be made below in conjunction with the accompanying drawings and specific embodiments.
[0063] The invention provides a long-term prediction method of network traffic based on deep learning. Aiming at the temporal correlation and spatial correlation of dynamic changes in network traffic, and the long-term memory ability of historical flow sequences, which is especially important in long-term network traffic forecasting, the Transformer model is used to extract spatio-temporal features and model global dependencies. And in order to alleviate the problem of inconsistent distribution of training data and test data, an adaptive training mechanism is proposed, and the input data is selected adaptively according to the error value in the training process, so as to maintain the balance of training data and test data. Improve the accuracy of long-term traffic forec...
PUM
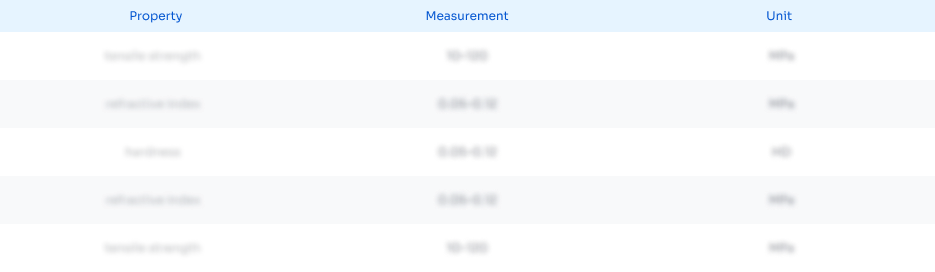
Abstract
Description
Claims
Application Information
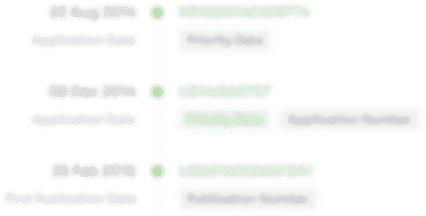
- R&D
- Intellectual Property
- Life Sciences
- Materials
- Tech Scout
- Unparalleled Data Quality
- Higher Quality Content
- 60% Fewer Hallucinations
Browse by: Latest US Patents, China's latest patents, Technical Efficacy Thesaurus, Application Domain, Technology Topic, Popular Technical Reports.
© 2025 PatSnap. All rights reserved.Legal|Privacy policy|Modern Slavery Act Transparency Statement|Sitemap|About US| Contact US: help@patsnap.com