Shared bicycle scheduling method based on deep reinforcement learning
A technology of reinforcement learning and scheduling methods, applied in the direction of neural learning methods, constraint-based CAD, design optimization/simulation, etc., can solve the problems of not considering the impact of supply and demand in the future time period, low travel volume, and affecting the supply and demand environment, etc., to achieve Reduce urban congestion and motor vehicle exhaust emissions, increase travel volume, and improve service quality
- Summary
- Abstract
- Description
- Claims
- Application Information
AI Technical Summary
Problems solved by technology
Method used
Image
Examples
Embodiment Construction
[0059] Embodiments of the present invention will be further described below in conjunction with the accompanying drawings.
[0060] Before describing the specific embodiments of the present invention, in order to make the scheme of the present invention more clear and complete, at first the abbreviations and key term definitions that appear in the present invention are explained:
[0061] OD traffic volume: refers to the traffic volume between the origin and destination. "O" comes from English ORIGIN, pointing out the starting point of the trip, and "D" comes from English DESTINATION, pointing out the destination of the trip.
[0062] MFMARL algorithm: Mean Field Multi-Agent Reinforcement Learning, a multi-agent reinforcement learning algorithm based on mean field game theory.
[0063] Such as figure 1 As shown, the present invention provides a kind of shared bicycle scheduling method based on deep reinforcement learning, comprising the following steps:
[0064] S1: Divide ...
PUM
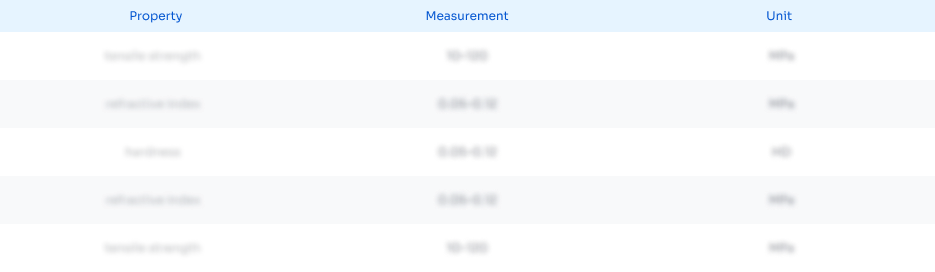
Abstract
Description
Claims
Application Information
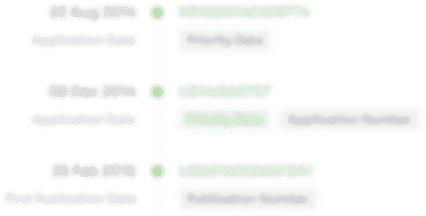
- R&D
- Intellectual Property
- Life Sciences
- Materials
- Tech Scout
- Unparalleled Data Quality
- Higher Quality Content
- 60% Fewer Hallucinations
Browse by: Latest US Patents, China's latest patents, Technical Efficacy Thesaurus, Application Domain, Technology Topic, Popular Technical Reports.
© 2025 PatSnap. All rights reserved.Legal|Privacy policy|Modern Slavery Act Transparency Statement|Sitemap|About US| Contact US: help@patsnap.com