Evaluation gene sets and kits for predicting the prognosis of liver cancer
A kit and gene set technology, applied in the field of evaluating the prognosis of liver cancer patients, can solve the problems of incomplete evaluation of prognosis and long observation time, and achieve the effect of reducing the limitation of mutation frequency and making accurate prediction
- Summary
- Abstract
- Description
- Claims
- Application Information
AI Technical Summary
Problems solved by technology
Method used
Image
Examples
Embodiment 1
[0053] Embodiment 1: Lasso regression method builds a model, obtains the selected characteristic gene set
[0054] Data processing, screening immune genes related to liver cancer prognosis
[0055] Download the gene expression data of hepatocellular carcinoma and clinical data such as patient overall survival time and survival endpoints from The Cancer Genome Atlas (TCGA), including 363 hepatocellular carcinoma samples, and the gene expression data contains 60483 genes. In order to construct a liver cancer prognosis prediction model, 547 immune-related genes were selected from 60483 genes for subsequent screening of gene sets for predicting patient prognosis.
[0056] Construction of Prognostic Model of Liver Cancer
[0057] Taking the clinical stage as a reference, 363 liver cancer patient samples in the TCGA dataset were randomly divided into 80% training set (290 samples) and 20% test set (73 samples). Using the training set samples and 547 immune-related genes, the l...
Embodiment 2
[0073] Example 2: Comparison of the predictive power of selected feature gene sets and random gene sets
[0074] In order to further verify the validity of the evaluation gene set of the selected 62 genes, other 62 genes were randomly selected from 547 genes (except the 62 genes selected above) to form a "random gene set", And compared with the selected "assessment gene set"; see Table 3 for the genes and their weight coefficients of the random gene set.
[0075] Table 3: The 62 genes of the random gene set and their weight coefficients
[0076] .
[0077] With reference to the process described in Example 1, patients are also divided into a training set (80%) and a test set (20%), and each gene in the random gene set in Table 3 and its weight coefficient are used to calculate the test set patients in this random model risk score in . The calculation method of the random gene set risk score is similar to that in Example 1. Calculate the C-index of the training set and th...
PUM
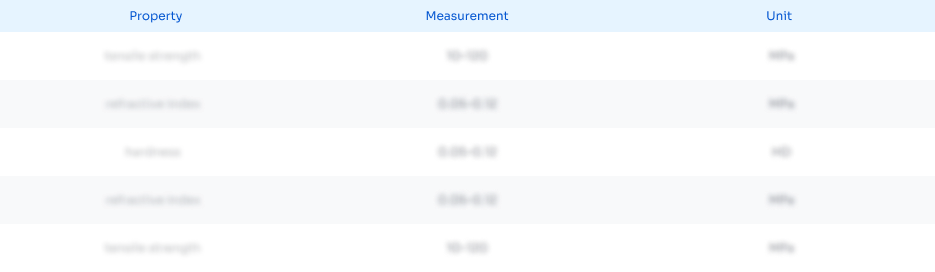
Abstract
Description
Claims
Application Information
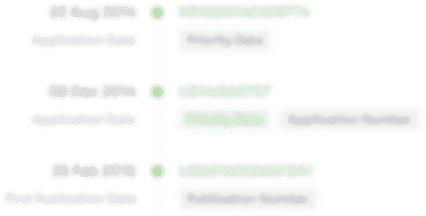
- R&D Engineer
- R&D Manager
- IP Professional
- Industry Leading Data Capabilities
- Powerful AI technology
- Patent DNA Extraction
Browse by: Latest US Patents, China's latest patents, Technical Efficacy Thesaurus, Application Domain, Technology Topic, Popular Technical Reports.
© 2024 PatSnap. All rights reserved.Legal|Privacy policy|Modern Slavery Act Transparency Statement|Sitemap|About US| Contact US: help@patsnap.com