Image classification method, system, medium and device during class distribution mismatching
A classification method and image technology, applied in the field of image processing, can solve the problems that classifiers have no positive effect, affect the accuracy of classification problems, affect the effect of model training, etc., achieve maximum utilization of labeling resources, wide application, and improve training effects Effect
- Summary
- Abstract
- Description
- Claims
- Application Information
AI Technical Summary
Problems solved by technology
Method used
Image
Examples
Embodiment 1
[0021] This embodiment discloses an image classification method when the class distribution does not match, such as including:
[0022] 1. Use the labeled images to train the initial image classification model and verify the image classification model.
[0023] The labeled images here and the unlabeled images below are all preprocessed images. Among them, preprocessing refers to the feature extraction of stored image data. Feature extraction methods include but not limited to directional gradient histogram, scale-invariant feature transformation, neural network feature extraction, etc., and then convert the feature-extracted image into a suitable The form for model training. Usually, the form suitable for model training refers to high-dimensional vectors that can reflect image features.
[0024] 2. If figure 2 As shown, the unlabeled images are selected from the verification results, and their query scores are obtained according to their semantic scores and information sco...
Embodiment 2
[0033] Based on the same inventive concept, this embodiment uses a specific example to describe the solution of Embodiment 1 in detail.
[0034] According to the subject of each image, the images are divided into different categories, for example, the images are divided into three categories: "cat", "dog" and "car". Assuming that during the training of the image classification model, the collected labeled images only contain two categories of "cat" and "dog", but the collected unlabeled images contain data of all three categories, the actual An image of class "car" is the data for which the class distribution does not match. The cycle training of the model is carried out according to the method in Embodiment 1. In each round of cycle, after the image classification model training is performed according to the current marked image, the query score of each item of data in the unlabeled image is calculated. Among them, samples whose real category is "car" will get a lower semant...
Embodiment 3
[0036] Based on the same inventive concept, this embodiment discloses an image classification system when the class distribution does not match, including:
[0037] A pre-training module, which uses marked images to train the initial image classification model and verify the image classification model;
[0038] The query scoring module is used to select unlabeled images from the verification results, obtain their query scores according to their semantic scores and information scores, sort them according to the query scores, and select images with high query scores according to the sorting results for labeling;
[0039] The secondary training module is used to add the marked image to the marked image, and train the initial image classification model until the training result meets the preset requirements;
[0040] An image classification module, which uses an image classification model meeting preset requirements to classify images.
PUM
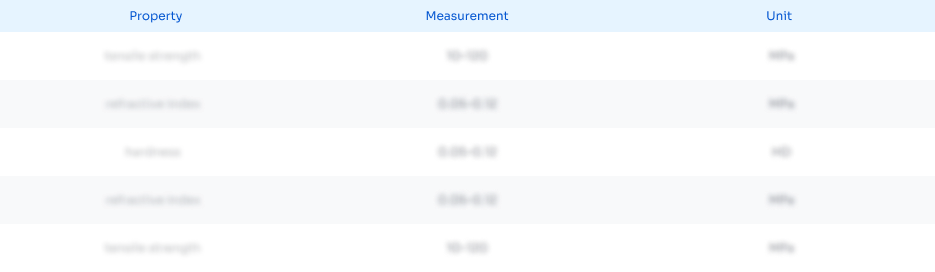
Abstract
Description
Claims
Application Information
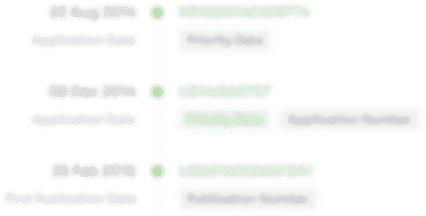
- R&D Engineer
- R&D Manager
- IP Professional
- Industry Leading Data Capabilities
- Powerful AI technology
- Patent DNA Extraction
Browse by: Latest US Patents, China's latest patents, Technical Efficacy Thesaurus, Application Domain, Technology Topic, Popular Technical Reports.
© 2024 PatSnap. All rights reserved.Legal|Privacy policy|Modern Slavery Act Transparency Statement|Sitemap|About US| Contact US: help@patsnap.com