Gradient Compression Method for Distributed DNN Training in Edge Computing Environment
A technology of edge computing and compression method, which is applied in the field of edge computing, can solve the problems of communication efficiency and poor optimization of model accuracy, and achieve the effect of reducing communication costs
- Summary
- Abstract
- Description
- Claims
- Application Information
AI Technical Summary
Problems solved by technology
Method used
Image
Examples
Embodiment Construction
[0047] Below in conjunction with specific embodiments, the present invention will be further illustrated, and it should be understood that these embodiments are only used to illustrate the present invention and not to limit the scope of the present invention. The modifications all fall within the scope defined by the appended claims of this application.
[0048]In this embodiment, an adaptive sparse ternary gradient compression method for distributed DNN training in a multi-edge computing environment. By establishing the selection criteria based on the number of gradients, designing a sparse threshold selection algorithm based on entropy, introducing gradient residuals and momentum correction to optimize the loss of model accuracy due to sparse compression, combining ternary gradient quantization and lossless coding techniques, effectively reducing edge distributed training Communication cost per iteration.
[0049] figure 1 This is an application scenario of the adaptive sp...
PUM
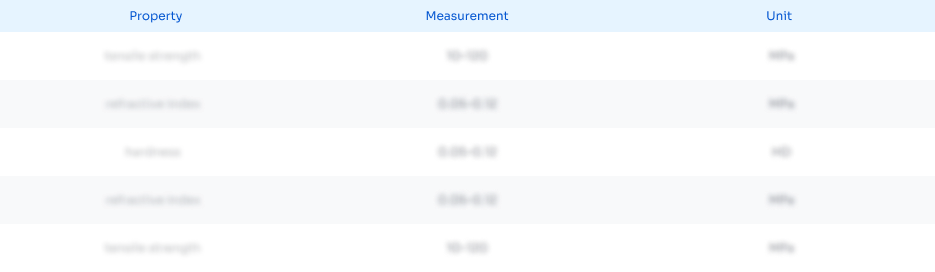
Abstract
Description
Claims
Application Information
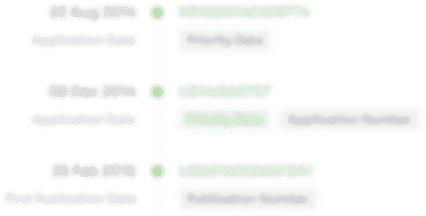
- R&D Engineer
- R&D Manager
- IP Professional
- Industry Leading Data Capabilities
- Powerful AI technology
- Patent DNA Extraction
Browse by: Latest US Patents, China's latest patents, Technical Efficacy Thesaurus, Application Domain, Technology Topic, Popular Technical Reports.
© 2024 PatSnap. All rights reserved.Legal|Privacy policy|Modern Slavery Act Transparency Statement|Sitemap|About US| Contact US: help@patsnap.com