Deep convolutional neural network model improvement method, system and device and medium
A neural network model and deep convolution technology, applied in the field of deep convolutional neural network models, can solve the problems of model parameter scale calculation and storage resource increase, application difficulty, and poor application effect, so as to reduce the amount of model parameters and calculation The effect of improving the speed of inference and reducing the amount of parameters
- Summary
- Abstract
- Description
- Claims
- Application Information
AI Technical Summary
Problems solved by technology
Method used
Image
Examples
Embodiment 1
[0055] Embodiment 1 provides a method for improving a deep convolutional neural network model, including steps:
[0056] Lightweighting the first deep convolutional neural network model to obtain a second deep convolutional neural network model;
[0057] Performing pruning processing on the second deep convolutional neural network model to obtain a third deep convolutional neural network model;
[0058] Using the third deep convolutional neural network model as a first student model and using the second deep convolutional neural network model as a first teacher model, perform knowledge distillation to obtain a fourth deep convolutional neural network model;
[0059] The parameter accuracy of the fourth deep convolutional neural network model is reduced.
[0060] The method flow in the present embodiment one is as follows figure 1 As shown, the lightweight improvement and compression of the convolution-based deep neural network model are carried out. The overall improvement ...
Embodiment 2
[0077] On the basis of Embodiment 1, when the deep convolutional neural network model is a DenseNet network model, that is, the deep convolutional neural network model is a DenseNet network model for the target classification and recognition task, at this time the DenseNet network model is adopted using the embodiment The lightweight method of the method in one is improved for lightweight. Experimental verification is carried out on the miniImageNet image dataset (100 categories, 60,000 color pictures, size 224x224), and the experimental results are shown in Table 1.
[0078] Table 1 DenseNet network model lightweight improvement and compression implementation results
[0079]
[0080]
[0081] Among them, in Table 1, Model is the model, and Metric is the measurement class library, which is a measurement class library for monitoring indicators. Table 1 involves three models for comparison, including the DenseNet-Baseline model, which is the DenseNet reference model, and ...
Embodiment 3
[0094] On the basis of Embodiment 1, when the deep convolutional neural network model is the Yolo-V4 network model, the lightweight method proposed by the present invention is used to improve the weight of the YOLO-V4 network. Experimental verification is carried out on the Pascal VOC2007-2012 image target detection dataset (20 categories, 21503 color pictures). The experimental results are shown in Table 4.
[0095] Table 4
[0096]
[0097] Among them, Top-1mAP is the mAP parameter index, representing the accuracy of the model, YOLOv4 (Bb-CSPDarknet53) is the original deep neural network, YOLOv4-DSC (Bb-CSPDarknet53) is the artificially designed improved network, among them, YOLOv4-DSC ( The left column of the Bb-CSPDarknet53) column is the parameters of the YOLOv4-DSC (Bb-CSPDarknet53) model, and the right column is the parameter comparison result of the YOLOv4-DSC (Bb-CSPDarknet53) model and the YOLOv4 (Bb-CSPDarknet53) model; from Table 4 It can be seen that the amoun...
PUM
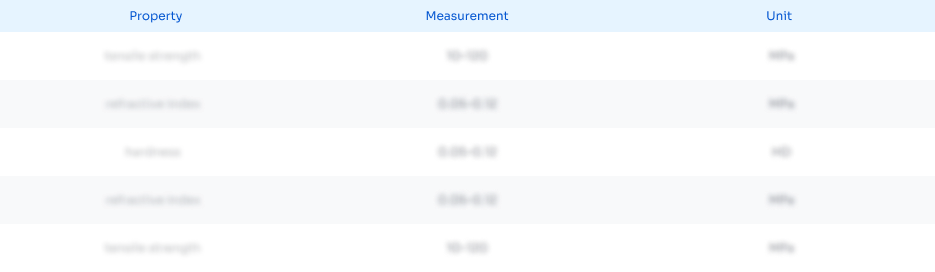
Abstract
Description
Claims
Application Information
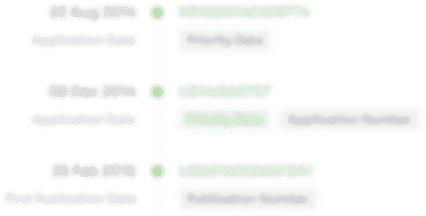
- R&D
- Intellectual Property
- Life Sciences
- Materials
- Tech Scout
- Unparalleled Data Quality
- Higher Quality Content
- 60% Fewer Hallucinations
Browse by: Latest US Patents, China's latest patents, Technical Efficacy Thesaurus, Application Domain, Technology Topic, Popular Technical Reports.
© 2025 PatSnap. All rights reserved.Legal|Privacy policy|Modern Slavery Act Transparency Statement|Sitemap|About US| Contact US: help@patsnap.com