Intelligent factory production job scheduling method and system based on deep reinforcement learning
A technology of reinforcement learning and job scheduling, applied in neural learning methods, machine learning, manufacturing computing systems, etc., can solve the problems of scheduling execution time growth, local optimization, etc., and achieve the effect of fast and efficient scheduling
- Summary
- Abstract
- Description
- Claims
- Application Information
AI Technical Summary
Problems solved by technology
Method used
Image
Examples
Embodiment Construction
[0043] The present invention will be further described below in conjunction with the accompanying drawings and embodiments.
[0044] Please refer to figure 1 , the present invention provides a method for scheduling production operations in an intelligent factory based on deep reinforcement learning, comprising the following steps:
[0045] Step S1: Computing and decomposing the production task data in the past or simulated generation in the cloud, obtaining the processing time of each task and each process on the corresponding machine, and forming a training set after preprocessing;
[0046] Step S2: build depth reinforcement learning DQN model, described depth reinforcement learning DQN model comprises DQN deep learning network structure and DQN reinforcement learning module;
[0047] Step S3: training depth reinforcement learning DQN model, obtains the depth reinforcement learning DQN model after training;
[0048] Step S4: input the pre-processed production task schedulin...
PUM
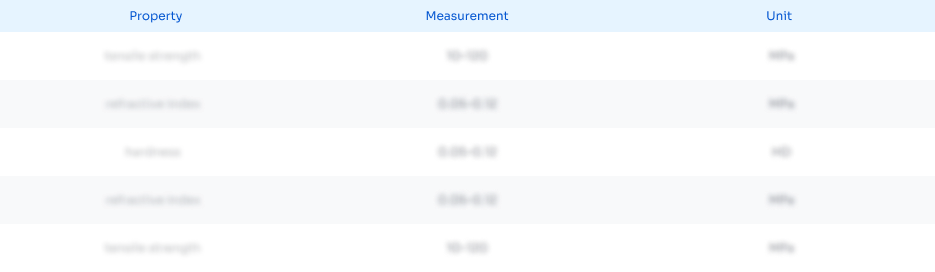
Abstract
Description
Claims
Application Information
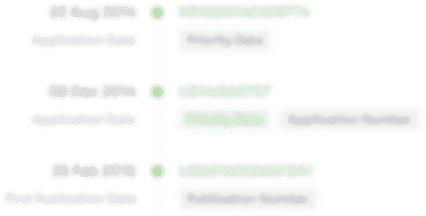
- R&D Engineer
- R&D Manager
- IP Professional
- Industry Leading Data Capabilities
- Powerful AI technology
- Patent DNA Extraction
Browse by: Latest US Patents, China's latest patents, Technical Efficacy Thesaurus, Application Domain, Technology Topic, Popular Technical Reports.
© 2024 PatSnap. All rights reserved.Legal|Privacy policy|Modern Slavery Act Transparency Statement|Sitemap|About US| Contact US: help@patsnap.com