Using image augmentation with simulated objects for training machine learning models
A technology for simulating objects and images, applied in the field of training machine learning models, can solve problems such as difficult to generate a practical, stable and reliable automatic driving system, and achieve the effect of improving decision-making results, increasing accuracy, and reducing high-precision time
- Summary
- Abstract
- Description
- Claims
- Application Information
AI Technical Summary
Problems solved by technology
Method used
Image
Examples
example 204
[0070] Figure 8 Is a flowchart of a method 800 for training a machine learning model using real world images augmented with simulated objects to detect objects in the images according to some embodiments of the present disclosure. At block B802, method 800 includes generating a first image of a virtual instance of an object from a virtual sensor perspective of a virtual vehicle in a virtual environment. For example, from the perspective of virtual sensors installed on a virtual vehicle in a simulated environment, a game-based engine such as Figure 2A Virtual instances 204, 206 and 208 of dead animals are shown.
[0071] At block B804, the method 800 includes determining a boundary shape corresponding to the virtual instance of the object. For example, a boundary shape (such as boundary shape 304 ) corresponding to a virtual instance (such as virtual instance 206 ) can be created using segmentation mask 214 associated with virtual instance 206 . In some embodiments, a boun...
PUM
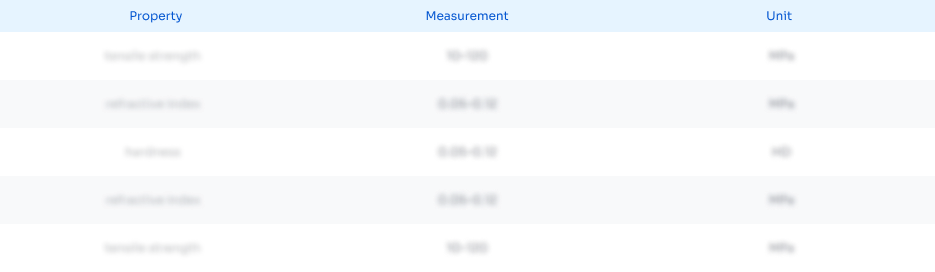
Abstract
Description
Claims
Application Information
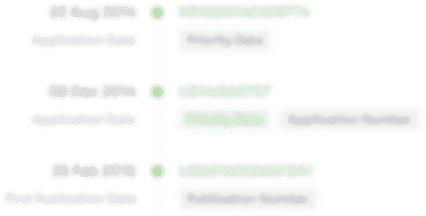
- R&D Engineer
- R&D Manager
- IP Professional
- Industry Leading Data Capabilities
- Powerful AI technology
- Patent DNA Extraction
Browse by: Latest US Patents, China's latest patents, Technical Efficacy Thesaurus, Application Domain, Technology Topic, Popular Technical Reports.
© 2024 PatSnap. All rights reserved.Legal|Privacy policy|Modern Slavery Act Transparency Statement|Sitemap|About US| Contact US: help@patsnap.com