Semantic segmentation model training method and device, electronic equipment and storage medium
A semantic segmentation and training method technology, applied in the field of deep learning, can solve the problems of unfavorable semantic segmentation model training and high labeling costs, and achieve the effect of good weak supervision model performance
- Summary
- Abstract
- Description
- Claims
- Application Information
AI Technical Summary
Problems solved by technology
Method used
Image
Examples
no. 1 example
[0076] Such as figure 2 As shown, the training method of a semantic segmentation model provided by the embodiment of the present application includes:
[0077] Step 201: Construct an image training set of sparse point annotations for semantic segmentation model training, including image I and its corresponding sparse point labels where |Y| is the number of sparse point labels corresponding to the image. Each sparse point label f includes a two-dimensional coordinate (h k ,w k ) to describe the position of the point, and a class label L k The class used to describe the point. An image can contain more than one arbitrary number of sparse point labels, such as image 3 shown;
[0078] Step 202: Construct a semantic segmentation model, which can adopt any deep model architecture based on gradient backpropagation for parameter update and learning. In this embodiment, the semantic segmentation model used for training includes a basic network model f=F(I; θ f ), used to m...
no. 2 example
[0087] In this embodiment, optionally, the step 204 in the above embodiment can also calculate the superpixel point set according to the original input image I Among them, |R| is the number of superpixels, each superpixel r i is a containing|r i |A collection of pixels According to the output prediction s of the semantic segmentation model in step 202, the category of each superpixel is calculated according to formula 4, and then the dense pixel-level pseudo-label is obtained, and the pseudo-label is used in combination with the segmentation loss function for model training, where Y'( r i ) for r i the category to which it belongs;
[0088]
[0089] In this embodiment, optionally, step 205 in the above embodiment can also be performed according to formula 3 using contrastive learning according to the middle layer feature expression e of the semantic segmentation model in step 202, combined with the pixel-level pseudo-label obtained in step 204 Model training, the par...
PUM
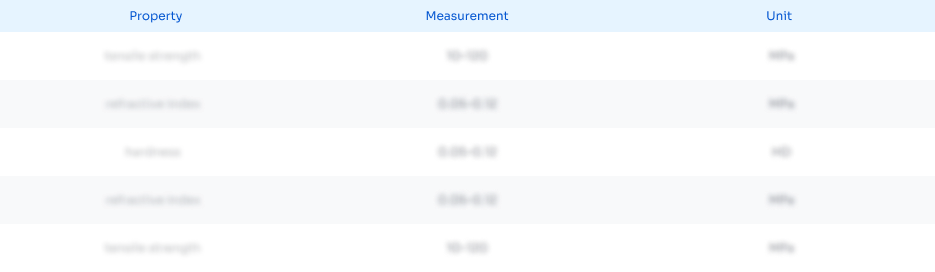
Abstract
Description
Claims
Application Information
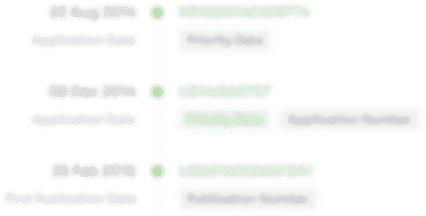
- R&D Engineer
- R&D Manager
- IP Professional
- Industry Leading Data Capabilities
- Powerful AI technology
- Patent DNA Extraction
Browse by: Latest US Patents, China's latest patents, Technical Efficacy Thesaurus, Application Domain, Technology Topic, Popular Technical Reports.
© 2024 PatSnap. All rights reserved.Legal|Privacy policy|Modern Slavery Act Transparency Statement|Sitemap|About US| Contact US: help@patsnap.com