Forestry pest identification method and system based on feature selection module
A feature selection and identification method technology, applied in the field of computer vision, can solve the problems of large differences in size and angle, low identification accuracy, and large changes in pest background information, so as to expand pest species, improve identification accuracy, and improve intelligent management. horizontal effect
- Summary
- Abstract
- Description
- Claims
- Application Information
AI Technical Summary
Problems solved by technology
Method used
Image
Examples
Embodiment 1
[0038] The present disclosure provides a method for identifying forestry pests based on a feature selection module, including:
[0039] Obtain images of forestry pests;
[0040] According to the obtained forestry pest image and the preset forestry pest identification network model, the forestry pest classification result is obtained, and the forestry pest identification is carried out;
[0041] Wherein, in the present embodiment, the ResNet feature extraction network selects the ResNet50 network, and the forestry pest recognition network model is obtained by expanding the forestry pest image after processing and the ResNet50 network training embedded with the feature selection module; the feature selection module is obtained by feature selection Units are combined with residual maps.
[0042] The ResNet residual network is a popular feature extraction network in recent years. The network creatively proposes a residual mapping structure, which effectively solves the problem of...
Embodiment 2
[0055] Such as figure 1 As shown, the present disclosure provides a method for identifying forestry pests based on a feature selection module, including:
[0056] 1. Obtain pictures of forestry pests and classify them according to the types of pests. Specifically, divide the collected pictures into a training set and a test set at a ratio of 4:1. The training set is used to train model parameters, and the test set is used to test the model. Calculation of parameter accuracy;
[0057] 2. Expand the data images in the divided training set;
[0058] In this embodiment, operations such as random flipping, increasing contrast, random grayscale processing, vertical mirror transformation, and horizontal mirror transformation are respectively used for data expansion; preferably, the original picture is randomly flipped from -180° to 180°, and the original The contrast of the image, the contrast floating ratio is 0.1, each image generates a grayscale image with a probability of 0.025...
Embodiment 3
[0071] The present embodiment provides a forestry pest identification system based on a feature selection module, including a data acquisition module and a species identification module;
[0072] The data acquisition module is configured to: acquire images of forestry pests;
[0073] The type identification module is configured to: obtain the forestry pest classification result according to the obtained forestry pest image and the preset forestry pest identification network model, and perform forestry pest identification;
[0074] Wherein, the forestry pest identification network model is obtained by expanding the processed forestry pest image training set and training the ResNet50 network embedded with the feature selection module; the feature selection module is obtained by combining the feature selection unit with the residual mapping.
PUM
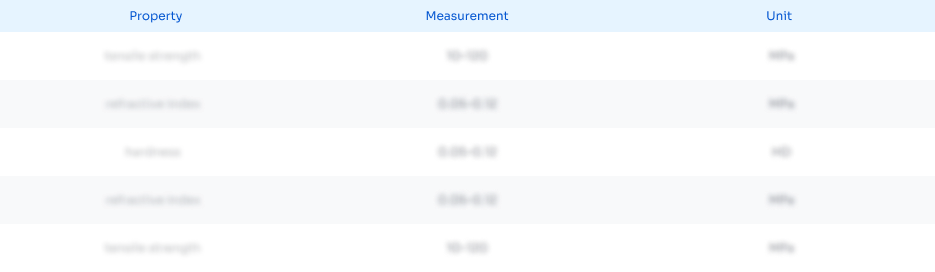
Abstract
Description
Claims
Application Information
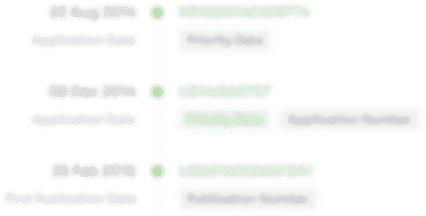
- R&D Engineer
- R&D Manager
- IP Professional
- Industry Leading Data Capabilities
- Powerful AI technology
- Patent DNA Extraction
Browse by: Latest US Patents, China's latest patents, Technical Efficacy Thesaurus, Application Domain, Technology Topic, Popular Technical Reports.
© 2024 PatSnap. All rights reserved.Legal|Privacy policy|Modern Slavery Act Transparency Statement|Sitemap|About US| Contact US: help@patsnap.com