Student score prediction method based on hypergraph neural network
A technology of neural network and prediction method, which is applied in the field of student performance prediction technology based on multi-source hypergraph neural network, can solve the problems of inability to accurately predict academic performance, inability to analyze students' multi-source heterogeneous behavior data, etc., and achieve good prediction accuracy rate, performance-enhancing effects
- Summary
- Abstract
- Description
- Claims
- Application Information
AI Technical Summary
Problems solved by technology
Method used
Image
Examples
Embodiment Construction
[0031] The present invention will be further described below in conjunction with the accompanying drawings.
[0032] 1. Model framework
[0033] The framework of the multi-source hypergraph neural network method is attached figure 1shown. The input of the model is a multi-source behavior matrix constructed by different behavior patterns of students (including learning, surfing the Internet, dining, shopping, showering, etc., including the content described above, but not limited to this.). The multi-source behavior matrix is divided and processed into multiple single-behavior matrices (learning matrix, dining matrix, etc.) according to the behavior pattern. The single-behavior matrix performs feature sensitivity analysis separately, and the features with high impact factors are screened out, and the features with low impact factors are deleted. The characteristics obtained after sensitivity analysis are called influence characteristics, and the matrix is called influenc...
PUM
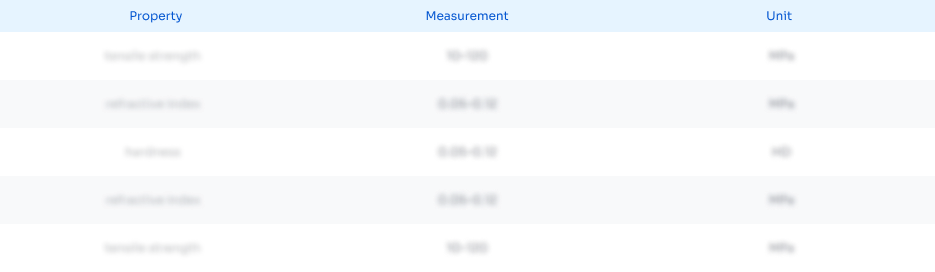
Abstract
Description
Claims
Application Information
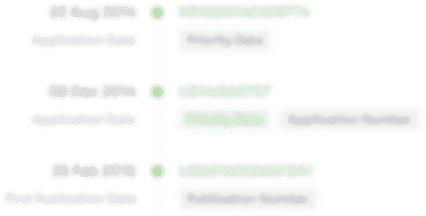
- Generate Ideas
- Intellectual Property
- Life Sciences
- Materials
- Tech Scout
- Unparalleled Data Quality
- Higher Quality Content
- 60% Fewer Hallucinations
Browse by: Latest US Patents, China's latest patents, Technical Efficacy Thesaurus, Application Domain, Technology Topic, Popular Technical Reports.
© 2025 PatSnap. All rights reserved.Legal|Privacy policy|Modern Slavery Act Transparency Statement|Sitemap|About US| Contact US: help@patsnap.com