Image segmentation method and device, equipment and storage medium
An image segmentation and image technology, applied in the field of artificial intelligence, can solve the problems of image difference, excessive labor and cost requirements, and low efficiency, and achieve the effect of reducing labor and cost, and improving the efficiency and accuracy of image segmentation.
- Summary
- Abstract
- Description
- Claims
- Application Information
AI Technical Summary
Problems solved by technology
Method used
Image
Examples
Embodiment approach 1
[0139] Embodiment 1: A discriminator is used to determine a first probability that a source-domain predicted sample image is a source-domain image, and a second probability that a target-domain predicted sample image is a target-domain image. Then, based on the first probability and the second probability, determine the adversarial learning loss value between the source domain image segmentation network to be trained, the target domain image segmentation network to be trained, and the discriminator. Based on the source label and the source predicted label, the segmentation loss value of the source domain image segmentation network to be trained is determined. Based on the adversarial learning loss value and the segmentation loss value, the target loss value is obtained.
[0140] Specifically, the neural network architecture involved in the training process is as Figure 7 As shown, it includes a source domain image segmentation network 501 to be trained, a target domain image...
Embodiment approach 2
[0145] Embodiment 2: A discriminator is used to determine the first probability that the predicted sample image in the source domain is an image in the source domain, and the second probability that the predicted sample image in the target domain is an image in the target domain. Then, based on the first probability and the second probability, determine the adversarial learning loss value between the source domain image segmentation network to be trained, the target domain image segmentation network to be trained, and the discriminator. Based on the source label and the source predicted label, the segmentation loss value of the source domain image segmentation network to be trained is determined. Based on the network parameters of the source domain classifier to be trained and the network parameters of the target domain classifier to be trained, the distance loss value between the source domain classifier to be trained and the target domain classifier to be trained is determine...
Embodiment approach 3
[0149] Embodiment 3: Determine the maximum average difference between the predicted sample image in the source domain and the predicted sample image in the target domain. Then, based on the source labels and source prediction labels, the segmentation loss value of the source domain image segmentation network to be trained is determined. Based on the adversarial learning loss value and the segmentation loss value, the target loss value is obtained.
[0150]Specifically, through the Maximum Mean Discrepancy (MMD) algorithm, the maximum mean difference between the predicted sample image and the target domain predicted sample image is determined, and the maximum mean difference is used to measure the distance between two different but related distributions . Based on the difference between the source label and the source prediction label, the segmentation loss value of the source domain image segmentation network to be trained is determined, and then the weighted sum between the ...
PUM
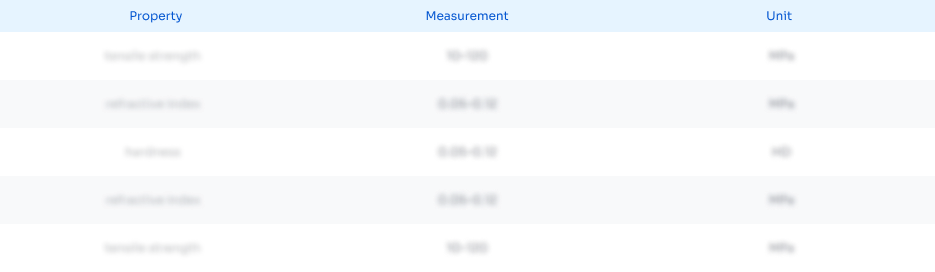
Abstract
Description
Claims
Application Information
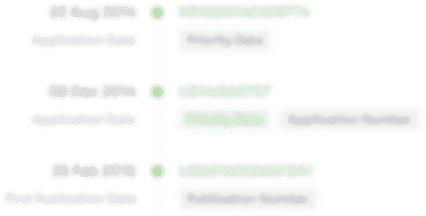
- R&D Engineer
- R&D Manager
- IP Professional
- Industry Leading Data Capabilities
- Powerful AI technology
- Patent DNA Extraction
Browse by: Latest US Patents, China's latest patents, Technical Efficacy Thesaurus, Application Domain, Technology Topic, Popular Technical Reports.
© 2024 PatSnap. All rights reserved.Legal|Privacy policy|Modern Slavery Act Transparency Statement|Sitemap|About US| Contact US: help@patsnap.com