Self-adaptive Rulkov neuron clustering method
A clustering method and neuron technology, applied in the field of pattern recognition, can solve the problems of small distance between classes, poor separability, and poor clustering effect, and achieve the effect of excellent clustering performance and good clustering effect.
- Summary
- Abstract
- Description
- Claims
- Application Information
AI Technical Summary
Problems solved by technology
Method used
Image
Examples
Embodiment 1
[0143] In this embodiment, the adaptive Rulkov neuron clustering method uses adaptive distance and shared neighbors to construct a similarity matrix, which can avoid the problem of difficult selection of scale parameters in spectral clustering algorithms based on normalized Laplacian matrices. The present invention uses a clustering method based on Rulkov neuron self-learning for clustering, and further studies the similarity between samples to increase the distance between samples. It can avoid the problem of poor clustering effect caused by improper selection of the initial clustering center when the spectral clustering algorithm based on the normalized Laplacian matrix uses the K-means algorithm. The flow chart of the adaptive Rulkov neuron clustering method is as follows: figure 1 shown.
[0144] The specific implementation manner of the present invention will be described in further detail below in conjunction with the accompanying drawings and specific examples.
[014...
PUM
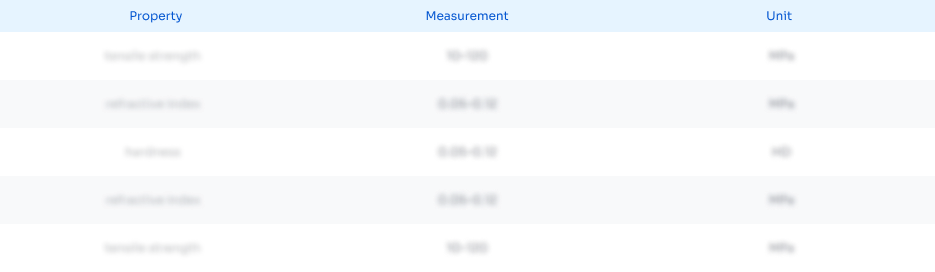
Abstract
Description
Claims
Application Information
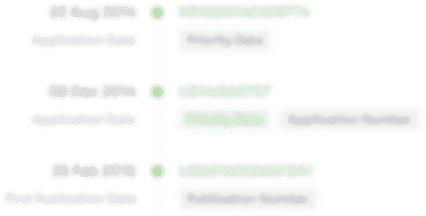
- R&D Engineer
- R&D Manager
- IP Professional
- Industry Leading Data Capabilities
- Powerful AI technology
- Patent DNA Extraction
Browse by: Latest US Patents, China's latest patents, Technical Efficacy Thesaurus, Application Domain, Technology Topic, Popular Technical Reports.
© 2024 PatSnap. All rights reserved.Legal|Privacy policy|Modern Slavery Act Transparency Statement|Sitemap|About US| Contact US: help@patsnap.com