Convolution kernel pruning model compression method and device
A compression method and convolution kernel technology, applied in the field of convolutional neural network model compression, can solve the problems of unimportant convolution kernel and insufficient clipping strength
- Summary
- Abstract
- Description
- Claims
- Application Information
AI Technical Summary
Problems solved by technology
Method used
Image
Examples
Embodiment Construction
[0028] The technical solutions in the embodiments of the present application will be clearly and completely described below in conjunction with the accompanying drawings in the embodiments of the present application. Obviously, the described embodiments are only some of the embodiments of the present application, not all of them. Based on the embodiments in this application, all other embodiments obtained by persons of ordinary skill in the art without creative efforts fall within the protection scope of this application.
[0029] The present invention will be further described in detail below through specific embodiments in conjunction with the accompanying drawings.
[0030] A convolution kernel pruning model compression method, the method flow is as follows: the first step: for the use scene, use the commonly used model training method to train a convolutional neural network to convergence; the second step: for the convolution layer All feature maps calculate their correspo...
PUM
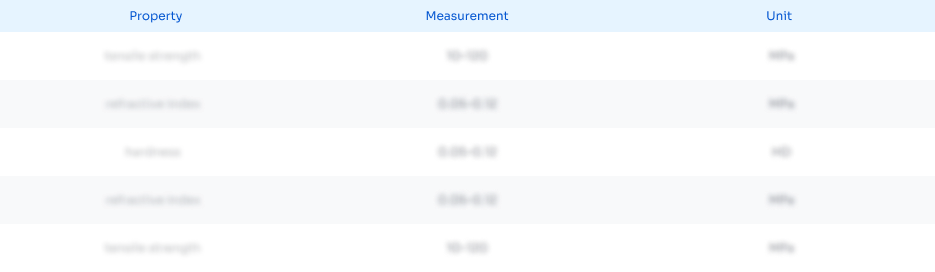
Abstract
Description
Claims
Application Information
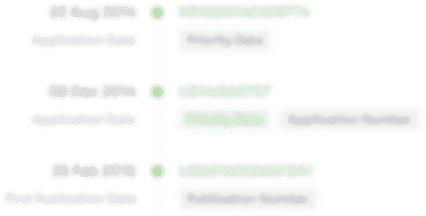
- R&D Engineer
- R&D Manager
- IP Professional
- Industry Leading Data Capabilities
- Powerful AI technology
- Patent DNA Extraction
Browse by: Latest US Patents, China's latest patents, Technical Efficacy Thesaurus, Application Domain, Technology Topic, Popular Technical Reports.
© 2024 PatSnap. All rights reserved.Legal|Privacy policy|Modern Slavery Act Transparency Statement|Sitemap|About US| Contact US: help@patsnap.com