Self-supervised learning method and self-supervised learning device
A supervised learning and covariance technology, applied in the computer field, can solve problems such as lack of semantic invariance, semantic deviation of augmented images, performance impact of computer vision tasks, etc.
- Summary
- Abstract
- Description
- Claims
- Application Information
AI Technical Summary
Problems solved by technology
Method used
Image
Examples
Embodiment Construction
[0041] The following will clearly and completely describe the technical solutions in the embodiments of the present disclosure with reference to the drawings in the embodiments of the present disclosure.
[0042] Unless otherwise specified, descriptions such as "first" and "second" in the present disclosure are used to distinguish different objects, and are not used to indicate meanings such as size or timing.
[0043] By flipping, panning, adding noise, cutting, etc., the original image can be processed to create various augmented images of the original image, which can be used as training samples for self-supervised learning. The augmentation algorithm may, for example, use a SwAV (SwappingAssignments between multiple Views of the same images, swapping assignments between multiple views of the same image) algorithm.
[0044] According to traditional self-supervised learning, it is assumed that these augmented images of the same original image have the consistency of semantic...
PUM
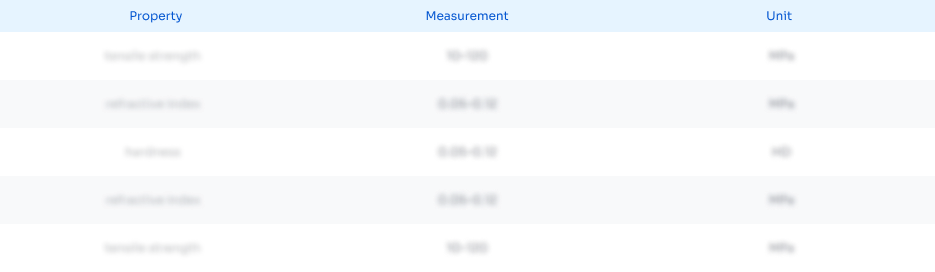
Abstract
Description
Claims
Application Information
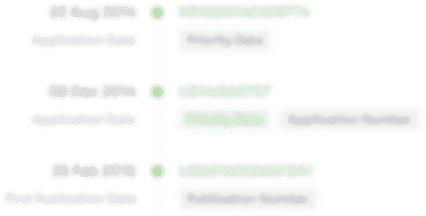
- R&D Engineer
- R&D Manager
- IP Professional
- Industry Leading Data Capabilities
- Powerful AI technology
- Patent DNA Extraction
Browse by: Latest US Patents, China's latest patents, Technical Efficacy Thesaurus, Application Domain, Technology Topic, Popular Technical Reports.
© 2024 PatSnap. All rights reserved.Legal|Privacy policy|Modern Slavery Act Transparency Statement|Sitemap|About US| Contact US: help@patsnap.com