Adaptive channel modeling method and system of enhanced conditional generative adversarial network
An adaptive channel and condition generation technology, applied in the field of digital communication, can solve problems such as difficulty in parameter analysis and lack of generality in modeling methods, and achieve the effect of improving system simulation performance and reducing the probability of non-convergence
- Summary
- Abstract
- Description
- Claims
- Application Information
AI Technical Summary
Problems solved by technology
Method used
Image
Examples
Embodiment 1
[0086] Such as figure 1 As shown, this embodiment enhances the adaptive channel modeling method of conditional generative adversarial network, and the specific steps are as follows:
[0087] (1) Steps of initializing the enhanced conditional generation confrontation network model
[0088] Step 1.1, set the generator model, including several layers of convolutional neural networks and single-layer variational neural networks; set the discriminator model, including several layers of deep neural networks and fully connected layers. Among them, each layer is mainly composed of fully connected layers (FC) Rectified Linear Unit (ReLU) layer, and the transfer function of a single FC-ReLU layer is:
[0089]
[0090] layer output value According to the input value weight vector bias vector Calculated layer output value At the same time the rectifier plays a non-linear role. where i represents the layer index, k represents the input index, and j represents the output index....
Embodiment 2
[0164] Such as Figure 7 As shown, the adaptive channel modeling system of the enhanced conditional generation confrontation network in this embodiment includes the following modules:
[0165] Initialize the module, initialize the generated confrontation network model, and obtain the channel sample sequence;
[0166] The prior knowledge sets the latent variable module, and the latent variable method is set through the prior knowledge to obtain the latent variable sequence;
[0167] The complex number sequence reconstruction module obtains the reconstructed sample sequence that meets the processing requirements of the neural network through the complex number sequence reconstruction method;
[0168] The penalty sample construction module obtains the penalty sample sequence through the penalty sample construction method;
[0169] The enhanced conditional generation confrontation network error function optimization module obtains the input sequence and the generation confrontat...
PUM
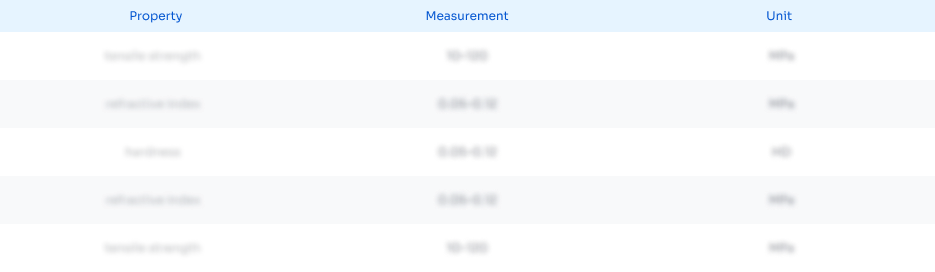
Abstract
Description
Claims
Application Information
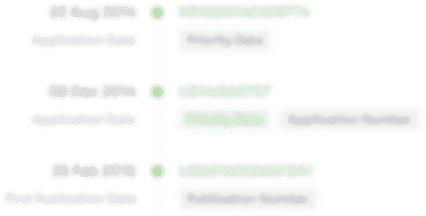
- R&D
- Intellectual Property
- Life Sciences
- Materials
- Tech Scout
- Unparalleled Data Quality
- Higher Quality Content
- 60% Fewer Hallucinations
Browse by: Latest US Patents, China's latest patents, Technical Efficacy Thesaurus, Application Domain, Technology Topic, Popular Technical Reports.
© 2025 PatSnap. All rights reserved.Legal|Privacy policy|Modern Slavery Act Transparency Statement|Sitemap|About US| Contact US: help@patsnap.com