Training method of battery state prediction model, and battery state prediction method and device
A battery status and prediction model technology, applied in the direction of measuring devices, measuring electricity, measuring electrical variables, etc., can solve problems such as limited computing power, inaccurate battery failure warning, and inability to accurately predict battery status, achieving the effect of improving accuracy
- Summary
- Abstract
- Description
- Claims
- Application Information
AI Technical Summary
Problems solved by technology
Method used
Image
Examples
Embodiment Construction
[0054] The present invention is further illustrated below by means of examples, but the present invention is not limited to the scope of the examples.
[0055] figure 1 It is a flowchart of a training method for a battery state prediction model provided by an exemplary embodiment of the present invention, the training method includes the following steps:
[0056] Step 101. Obtain measurement operation data and attribute data of the battery, and construct an electrochemical model of the battery according to the measurement operation data and attribute data.
[0057] The measurement operation data may be the data measured during the test process of charging and discharging the battery, preferably. The number of batteries can be one or more, that is, the electrochemical model of the battery is constructed based on the measurement operation data and attribute data of one battery, or the electrochemical model of the battery is constructed based on the measurement operation data an...
PUM
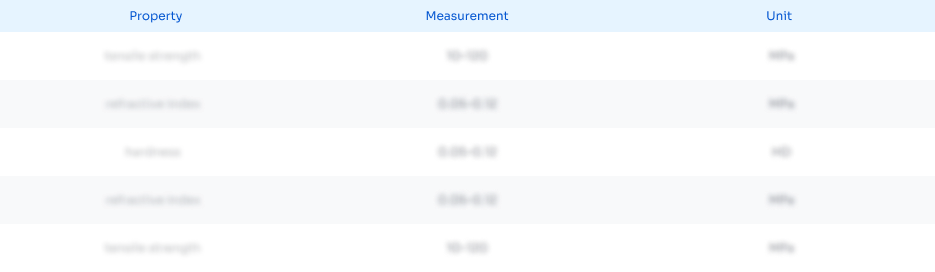
Abstract
Description
Claims
Application Information
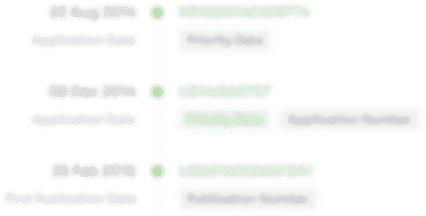
- R&D Engineer
- R&D Manager
- IP Professional
- Industry Leading Data Capabilities
- Powerful AI technology
- Patent DNA Extraction
Browse by: Latest US Patents, China's latest patents, Technical Efficacy Thesaurus, Application Domain, Technology Topic.
© 2024 PatSnap. All rights reserved.Legal|Privacy policy|Modern Slavery Act Transparency Statement|Sitemap