Riemannian manifold deep convolutional network image classification method
A deep convolution, network image technology, applied in neural learning methods, biological neural network models, instruments, etc., can solve problems such as information loss, performance not increasing but decreasing, and error increasing.
- Summary
- Abstract
- Description
- Claims
- Application Information
AI Technical Summary
Problems solved by technology
Method used
Image
Examples
Embodiment Construction
[0013] figure 1 It is the RM-CNN network structure diagram of the present invention; the mark in RM-CNN: COV refers to the covariance operation module, and AFL refers to the series connection of AvgPool2d and Flatten layers, and its function is to average pool down-sampling and straighten the two-dimensional matrix It is a one-dimensional vector; SAN is a self-attention module; add three modules of COV, AFL, and SAN to the output nodes of conv1, conv2_x, conv3_x, conv4_x, and conv5_x of ResNet in sequence. These three modules are connected in series and connected to the end of ResNet. The output features are directly spliced, and the length of the spliced features is the sum of all features; finally, two SAN modules are connected in series at the end of the network, and the first module is short-circuited;
[0014] Attached below figure 2 The inventive method is described further, and concrete implementation steps are as follows:
[0015] Step 1, pre-train RM-CNN on the l...
PUM
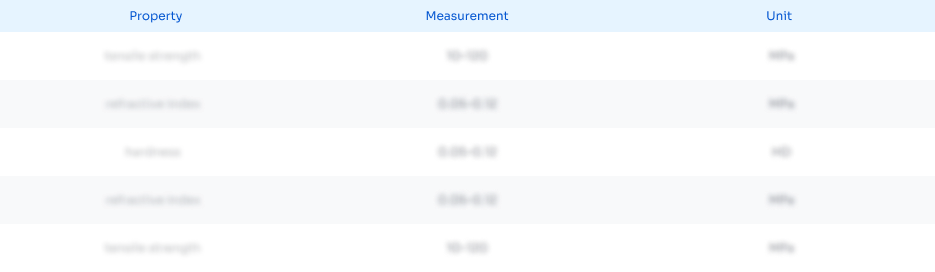
Abstract
Description
Claims
Application Information
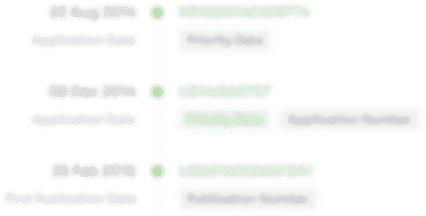
- R&D Engineer
- R&D Manager
- IP Professional
- Industry Leading Data Capabilities
- Powerful AI technology
- Patent DNA Extraction
Browse by: Latest US Patents, China's latest patents, Technical Efficacy Thesaurus, Application Domain, Technology Topic, Popular Technical Reports.
© 2024 PatSnap. All rights reserved.Legal|Privacy policy|Modern Slavery Act Transparency Statement|Sitemap|About US| Contact US: help@patsnap.com