A Cross-modal Feature Fusion System Based on Attention Mechanism
A feature fusion and cross-modal technology, applied in computer parts, character and pattern recognition, biological neural network models, etc., can solve problems that are not involved
- Summary
- Abstract
- Description
- Claims
- Application Information
AI Technical Summary
Problems solved by technology
Method used
Image
Examples
Embodiment 1
[0063] like figure 1 shown, a cross-modal feature fusion system based on attention mechanism, including:
[0064] Audio and video correlation analysis module, used to align the two modalities of audio and video RGB images;
[0065] A supervised contrastive learning module is used to extract modal features from two modalities of audio and video RGB images;
[0066] A cross-modal feature fusion module for learning global contextual representations by exploiting the relevant knowledge between modalities.
[0067] The audio-video correlation analysis module continuously collects 16 frames of RGB images from a video i to generate RGB segments v i As the input of RGB image mode; at this time, only one segment is sampled in a video, in order to make full use of the effective audio information in a video, the audio extracted from the entire video i is converted into the mel spectrogram of the video a i As the input of the audio modality; where, i=1,...,N.
[0068] The specific pr...
Embodiment 2
[0076] like figure 1 shown, a cross-modal feature fusion system based on attention mechanism, including:
[0077] Audio and video correlation analysis module, used to align the two modalities of audio and video RGB images;
[0078] A supervised contrastive learning module is used to extract modal features from two modalities of audio and video RGB images;
[0079] A cross-modal feature fusion module for learning global contextual representations by exploiting the relevant knowledge between modalities.
[0080] The audio-video correlation analysis module continuously collects 16 frames of RGB images from a video i to generate RGB segments v i As the input of RGB image mode; at this time, only one segment is sampled in a video, in order to make full use of the effective audio information in a video, the audio extracted from the entire video i is converted into the mel spectrogram of the video a i As the input of the audio modality; where, i=1,...,N.
[0081] The specific pr...
Embodiment 3
[0119] For the convenience of describing each module, given N different videos, the segment of each video consists of a size of where c is the number of channels, l is the number of frames, and h and w represent the height and width of the frame. The size of the 3D convolution kernel is t×d×d, where t is the time length and d is the spatial size; the video RGB image sequence is defined as , where v i An RGB segment generated for consecutively sampling m frames from a video i (i=1,...,N). The audio modality is the mel spectrogram generated by the short-time Fourier transform of the entire audio of a video; a segment of the video RGB image and the mel spectrogram generated by the entire video are aligned as input; the audio mel spectrogram sequence Expressed as , where a i A mel-spectrogram generated for audio extracted from a video i. is the category label for video i.
[0120] 1), audio and video correlation analysis (audio and video alignment)
[0121] The sound si...
PUM
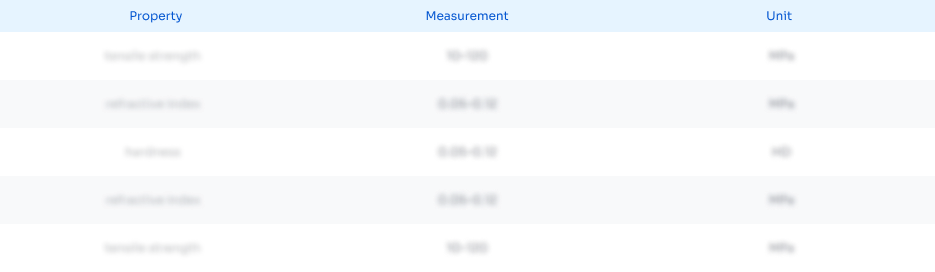
Abstract
Description
Claims
Application Information
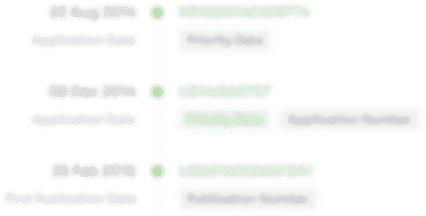
- R&D Engineer
- R&D Manager
- IP Professional
- Industry Leading Data Capabilities
- Powerful AI technology
- Patent DNA Extraction
Browse by: Latest US Patents, China's latest patents, Technical Efficacy Thesaurus, Application Domain, Technology Topic, Popular Technical Reports.
© 2024 PatSnap. All rights reserved.Legal|Privacy policy|Modern Slavery Act Transparency Statement|Sitemap|About US| Contact US: help@patsnap.com