Method for predicting residual life of avionics product
A life prediction and product technology, which is applied in prediction, neural learning methods, instruments, etc., can solve problems such as the dependence of the prediction effect on the degree of consistency, the definition of the remaining life of the real product, and the lack of learning ability to support it, so as to achieve good overall reusability , fast running and highly reusable effects
- Summary
- Abstract
- Description
- Claims
- Application Information
AI Technical Summary
Problems solved by technology
Method used
Image
Examples
Embodiment Construction
[0017] see figure 1 . According to the present invention, sensors are preset in avionics products, functional performance index data of similar products and real products are collected and recorded, static feature extraction and normalization processing are performed on the functional index data obtained by the preset sensors, and The functional performance index data is constructed as a sample set of similar products SP_SAMPLE and a sample set of real products RP_SAMPLE, and the construction of the sample set is completed;
According to different structural range parameters, initialize multiple LSTM time series models to form an LSTM model group with three gate structures of forget gate, input gate, and output gate and network units, and input similar product sample sets into the initialized LSTM model group to predict LSTM. Model group initial training and transfer training. In transfer learning training, the real product sample set is input into the LSTM model group after ...
PUM
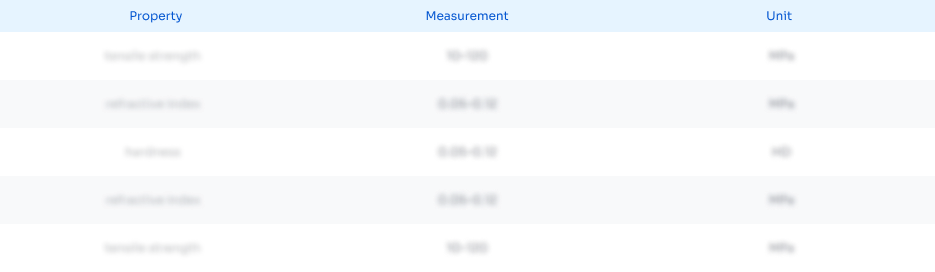
Abstract
Description
Claims
Application Information
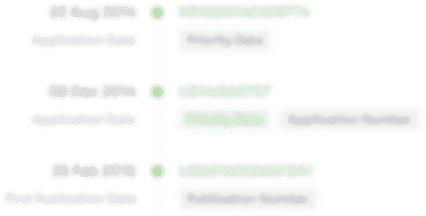
- R&D Engineer
- R&D Manager
- IP Professional
- Industry Leading Data Capabilities
- Powerful AI technology
- Patent DNA Extraction
Browse by: Latest US Patents, China's latest patents, Technical Efficacy Thesaurus, Application Domain, Technology Topic, Popular Technical Reports.
© 2024 PatSnap. All rights reserved.Legal|Privacy policy|Modern Slavery Act Transparency Statement|Sitemap|About US| Contact US: help@patsnap.com