Disease risk prediction method and system based on multi-source graph neural network fusion
A disease risk, neural network technology used in the medical field
- Summary
- Abstract
- Description
- Claims
- Application Information
AI Technical Summary
Problems solved by technology
Method used
Image
Examples
Embodiment 1
[0033] The present embodiment 1 provides a disease risk prediction system based on multi-source graph neural network fusion, including:
[0034] The disease risk prediction set and the disease relationship network building module, by obtaining the patient's diagnostic record data, standardizing the patient's medical record, and integrating the diagnosis results of the patient's multiple visits from the perspective of the duration of illness to construct a disease prediction data set. Construct multi-source disease relationship network, including: disease co-occurrence network based on comorbidity, disease relationship network based on disease coding and disease network based on phenotype-genotype relationship.
[0035]The disease risk prediction module uses the constructed disease risk prediction model based on multi-source graph neural network fusion to predict the disease risk of patients and obtain the final disease risk; among them, the disease risk prediction model first u...
Embodiment 2
[0046] In this Example 2, a method for data integration and construction of a multi-source disease relationship network is proposed, and three disease relationship networks are constructed based on three disease relationship data, namely the disease co-occurrence network based on comorbidities, and the disease co-occurrence network based on ICD-10. Disease relationship networks and disease networks based on phenotype-genotype relationships, such as figure 2 shown.
[0047] (1) Disease network based on co-occurrence of comorbid diseases
[0048] A patient suffers from one disease and often suffers from multiple other diseases. This section constructs a disease co-occurrence network through the co-occurrence of the patient's disease. Each node of the network represents a disease, and the edge represents that the diseases corresponding to the nodes at both ends have a co-occurrence relationship, and the weight is the number of patients suffering from both diseases in the medica...
Embodiment 3
[0060] In this embodiment 3, a disease risk prediction model based on multi-source graph neural network fusion is proposed to achieve high-precision prediction of disease risk.
[0061] Disease risk prediction models based on disease duration data (such as image 3 (shown) contains three modules, A module is to extract the patient's historical disease characteristics; B module learns the node feature matrix of multiple disease networks with the help of a graph convolutional neural network model, and then calculates the high-dimensional characteristics of patients based on the disease network; Finally, the C module splices the patient characteristics learned by the A module and the B module, and uses the multi-layer perceptron to predict the patient's risk of new diseases in the future, as follows.
[0062] (1) Patient clinical characteristics learning module A. This module mainly includes ICD adjacency matrix activation and convolutional neural network (CNN) feature extractio...
PUM
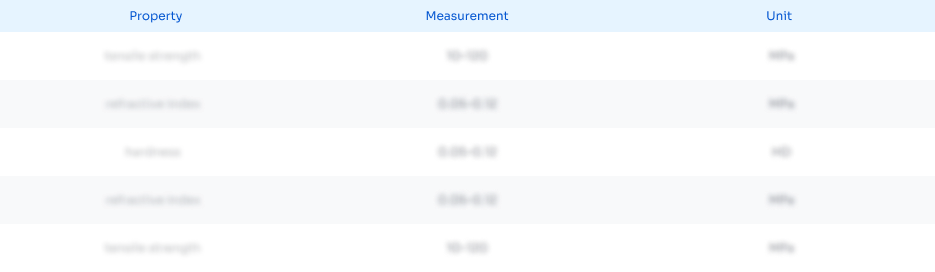
Abstract
Description
Claims
Application Information
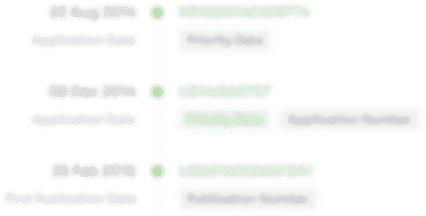
- R&D Engineer
- R&D Manager
- IP Professional
- Industry Leading Data Capabilities
- Powerful AI technology
- Patent DNA Extraction
Browse by: Latest US Patents, China's latest patents, Technical Efficacy Thesaurus, Application Domain, Technology Topic, Popular Technical Reports.
© 2024 PatSnap. All rights reserved.Legal|Privacy policy|Modern Slavery Act Transparency Statement|Sitemap|About US| Contact US: help@patsnap.com