Microgrid load prediction method based on long and short term neural network model
A neural network model, load forecasting technology, applied in biological neural network models, neural learning methods, forecasting, etc., to achieve the effect of improving convergence speed and stability, and improving forecasting accuracy
- Summary
- Abstract
- Description
- Claims
- Application Information
AI Technical Summary
Problems solved by technology
Method used
Image
Examples
Embodiment 1
[0043] Research shows that the microgrid load output is not only affected by the structure and characteristics of the system itself, but also by factors outside the system. From an economic point of view, the gross regional product (GDP) is closely related to the load output. From an industrial point of view, the proportion of electricity consumed by regional factories has a greater impact on the load. From the perspective of meteorological factors, especially extreme weather has a greater impact on the load output. From a time series perspective, time, month, season, and holidays all affect the load. From a market point of view, the value of peak and valley electricity will also affect the load. From the perspective of the microgrid structure, the proportion of new energy will also affect the load. In order to further reveal the influence of different types of influencing factors on the power generation load of the microgrid, the present invention conducts a characteristic...
Embodiment 2
[0084] In order to better understand the technical content of the present invention, this embodiment briefly summarizes the present invention through a system structure diagram, such as Figure 4As shown, the improved LSTM neural network proposed by the present invention. Through clustering algorithm and feature selection, the data set of key factors that have the greatest impact on the power generation load of the microgrid is obtained as the input of the network. Feature extraction is performed through two-layer convolutional neural network channels. The two convolutional layers exchange data through cross-training and other methods to further mine the high-value information contained in the data. The output features of the convolutional neural network are then used as the input of the LSTM. Its unique 3 gates and memory unit can further filter the features. At the same time, the convolutional neural network is inversely optimized using the Lookahead optimizer. Finally, ...
PUM
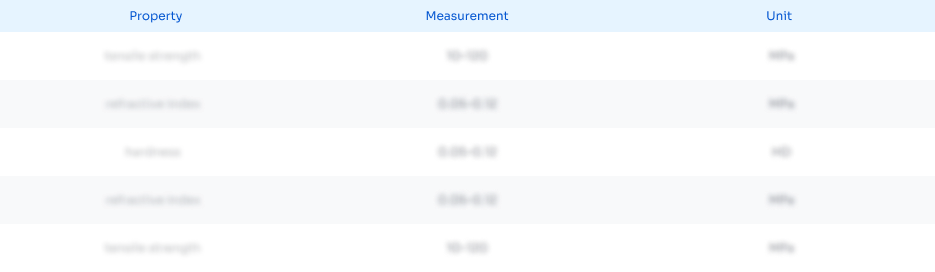
Abstract
Description
Claims
Application Information
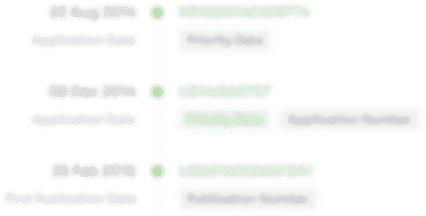
- Generate Ideas
- Intellectual Property
- Life Sciences
- Materials
- Tech Scout
- Unparalleled Data Quality
- Higher Quality Content
- 60% Fewer Hallucinations
Browse by: Latest US Patents, China's latest patents, Technical Efficacy Thesaurus, Application Domain, Technology Topic, Popular Technical Reports.
© 2025 PatSnap. All rights reserved.Legal|Privacy policy|Modern Slavery Act Transparency Statement|Sitemap|About US| Contact US: help@patsnap.com