Systems and methods for determining optimal parameters for dynamic quantum clustering analyses
a dynamic quantum and clustering analysis technology, applied in the field of data clustering, can solve the problems of data clustering that is often ill-defined in a mathematical sense, the performance of this quantum clustering approach can have an undetectable high sensitivity to the value of the quantum clustering scale parameter, and achieve the effect of reducing the dimensionality of the set of source data
- Summary
- Abstract
- Description
- Claims
- Application Information
AI Technical Summary
Benefits of technology
Problems solved by technology
Method used
Image
Examples
Embodiment Construction
[0055]Turning now to the drawings, systems and methods for dynamic quantum clustering in accordance with embodiments of the invention are described. Dynamic quantum clustering (DQC) can be flexibly applied to datasets that present significantly different challenges, we also show how a simple analysis can be used to look for the needle in the haystack, determine what it is, and find what this means. In this work, we advocate the use of a Schrödinger Hamiltonian Ĥ that is intimately connected to the data-structure, as defined by the quantum clustering method summarized below. We extend it into a time-dependent Schrödinger equation:
[0056]i∂ψ(x→,t)∂t=H^ψ(x→,t)(2)
The ensuing DQC formalism allows us, by varying a few parameters, to study in detail the temporal evolution of wave-functions representing the original data points. In turn, this dynamical behavior allows us to explore the structure of the quantum potential function defined by the quantum clustering method.
[0057]DQC begins ...
PUM
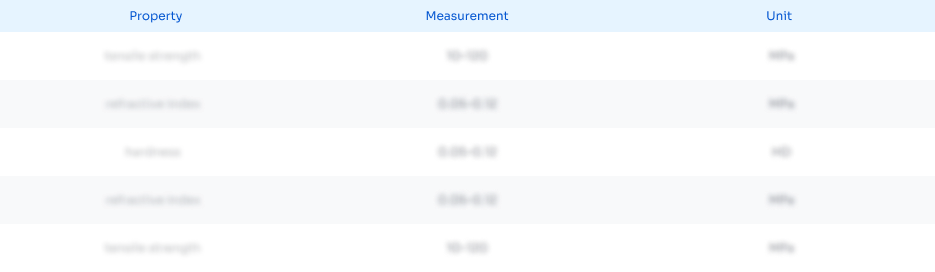
Abstract
Description
Claims
Application Information
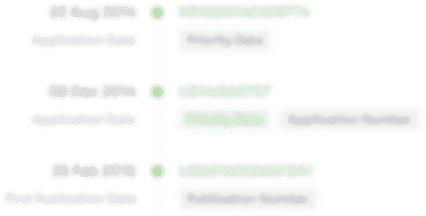
- R&D Engineer
- R&D Manager
- IP Professional
- Industry Leading Data Capabilities
- Powerful AI technology
- Patent DNA Extraction
Browse by: Latest US Patents, China's latest patents, Technical Efficacy Thesaurus, Application Domain, Technology Topic.
© 2024 PatSnap. All rights reserved.Legal|Privacy policy|Modern Slavery Act Transparency Statement|Sitemap