Hierarchical reinforcement learning task graph evolution method based on cause-and-effect diagram
A technology of reinforcement learning and task graph, applied in genetic models, instruments, electrical digital data processing, etc., can solve problems such as falling into local optimum, and achieve the effects of speeding up search, speeding up learning, and improving adaptability
- Summary
- Abstract
- Description
- Claims
- Application Information
AI Technical Summary
Problems solved by technology
Method used
Image
Examples
Embodiment Construction
[0050] The present invention will be described in detail below in conjunction with the accompanying drawings.
[0051] HI-MAT uses DBN on a successful trajectory of existing reinforcement learning tasks to construct the MAXQ task hierarchy, and then uses the constructed task graph on the target task. However, what HI-MAT obtains is a task structure diagram consistent with this trajectory, which is easy to fall into a local optimum. The present invention proposes a task graph evolution method based on causal graphs to construct a task graph more suitable for the target environment. This method mainly adjusts the search direction of the hierarchical space of the task graph according to the causal graph of the target environment, and maintains the causal dependence of the related state variables of the adjusted nodes in the task graph in the causal graph during the operation of the genetic operator. In the process, the adaptability of the task graph is improved, thereby speeding u...
PUM
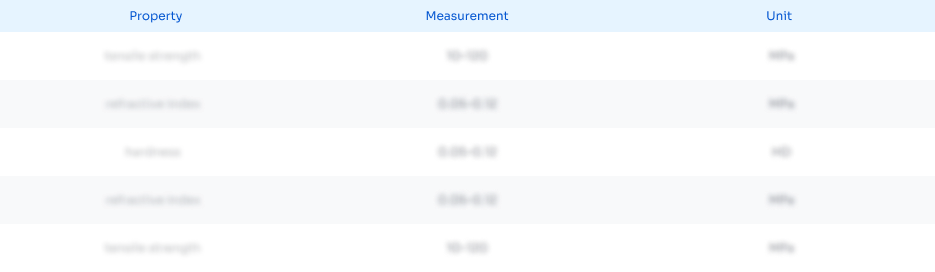
Abstract
Description
Claims
Application Information
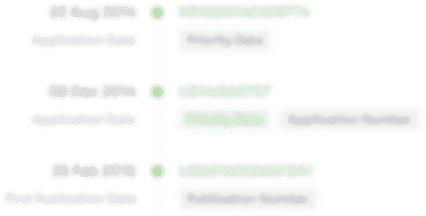
- R&D Engineer
- R&D Manager
- IP Professional
- Industry Leading Data Capabilities
- Powerful AI technology
- Patent DNA Extraction
Browse by: Latest US Patents, China's latest patents, Technical Efficacy Thesaurus, Application Domain, Technology Topic, Popular Technical Reports.
© 2024 PatSnap. All rights reserved.Legal|Privacy policy|Modern Slavery Act Transparency Statement|Sitemap|About US| Contact US: help@patsnap.com