Transfer learning method based on semi-supervised clustering
A semi-supervised clustering and transfer learning technology, applied in special data processing applications, instruments, electrical digital data processing, etc., can solve the problems of low accuracy, large classification error, and low efficiency of transfer learning process, and achieve accuracy High and efficient effect
- Summary
- Abstract
- Description
- Claims
- Application Information
AI Technical Summary
Problems solved by technology
Method used
Image
Examples
Embodiment Construction
[0019] The present invention adopts standard text data 20Newsgroup as experimental data. 20Newsgroup is a document set containing 20,000 newsgroups, divided into 20 subcategories and 7 top-level categories. In order to make the data set satisfy the migration scenario, the present invention reconstructs the data set. Since the dataset contains subcategories belonging to different top-level categories, subcategories of the same top-level category can be divided into subcategories of different top-level categories to form datasets in different fields. Based on this reconstruction, the source domain data and the target data come from different distributions, but because their subclasses have the same top-level class, there is a certain relationship between the two domain data. The source field and target field data are as follows:
[0020]
[0021]
[0022]
[0023] The present invention is described in detail below in conjunction with accompanying drawing example:
[...
PUM
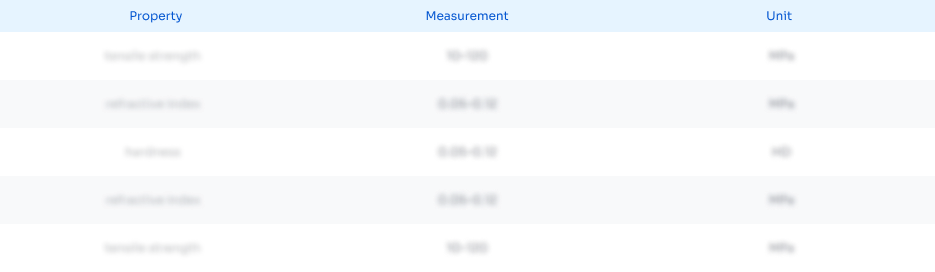
Abstract
Description
Claims
Application Information
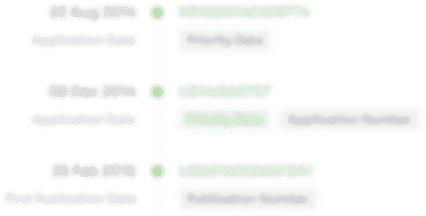
- R&D Engineer
- R&D Manager
- IP Professional
- Industry Leading Data Capabilities
- Powerful AI technology
- Patent DNA Extraction
Browse by: Latest US Patents, China's latest patents, Technical Efficacy Thesaurus, Application Domain, Technology Topic.
© 2024 PatSnap. All rights reserved.Legal|Privacy policy|Modern Slavery Act Transparency Statement|Sitemap