Gear fault diagnosis method based on improving multivariable predictive models
A variable prediction model and prediction model technology, which is applied in machine gear/transmission mechanism testing, character and pattern recognition, instruments, etc., can solve problems such as accuracy to be improved, data loss, and impact on classification accuracy, so as to reduce frequency confusion , effective analysis and processing, the real effect of instantaneous amplitude
- Summary
- Abstract
- Description
- Claims
- Application Information
AI Technical Summary
Problems solved by technology
Method used
Image
Examples
Embodiment Construction
[0039] The fault object in the embodiment of the present invention can be a gear, but is not limited to a gear. After reading the present invention, the gear fault diagnosis method based on the improved multivariate prediction model adopted by those skilled in the art according to the method of the present invention belongs to the protection scope of the present invention. In the specific embodiment, the gear is used as a preferred embodiment to explain the Fangming method, which is not a limiting condition of the present invention. In addition, the present invention does not require strict implementation of the sequence of steps in the following drawings, and any method that can achieve the purpose of fault diagnosis according to the above method belongs to the protection scope of the present invention.
[0040] see figure 1 , figure 1 is a flowchart of the present invention. Concrete implementation of the present invention comprises the steps:
[0041] Step S11, measuring...
PUM
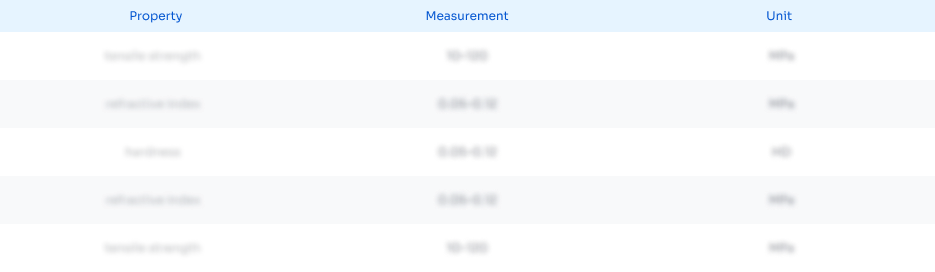
Abstract
Description
Claims
Application Information
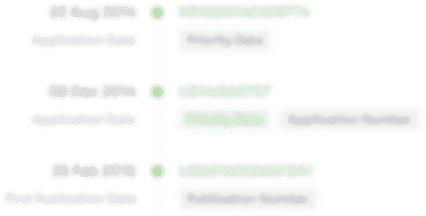
- R&D Engineer
- R&D Manager
- IP Professional
- Industry Leading Data Capabilities
- Powerful AI technology
- Patent DNA Extraction
Browse by: Latest US Patents, China's latest patents, Technical Efficacy Thesaurus, Application Domain, Technology Topic, Popular Technical Reports.
© 2024 PatSnap. All rights reserved.Legal|Privacy policy|Modern Slavery Act Transparency Statement|Sitemap|About US| Contact US: help@patsnap.com