Bayesian network-based rolling bearing fault diagnosis method
A Bayesian network and fault diagnosis technology, applied in mechanical bearing testing and other directions, can solve complex problems, achieve outstanding fault characteristics, improve accuracy and speed, and good interpretability
- Summary
- Abstract
- Description
- Claims
- Application Information
AI Technical Summary
Problems solved by technology
Method used
Image
Examples
Embodiment Construction
[0040] The present invention will be described in detail below in combination with specific embodiments.
[0041] Step 1: Set the fault diagnosis reliability threshold parameter θ * and fault sample initial parameters. Set the size of the initial value of the sample data group m; set the number of fault type Bearing value event q; set the fault type initial value parameter s={1,...,q}, type tag tag_s={1,...,q}. θ * The range is generally 0.7 to 0.8 (ie 70% to 80%); the value of m is usually 80 to 100; the value of q is usually 3 or 4.
[0042] Step 2: The vibration signal of the bearing is often monitored and collected through the vibration sensor system installed in the bearing seat, box, etc., and the vibration signal caused by different faults is sampled to obtain sample data data_s={tag_s f s (n) | L=mN; m and N are positive natural numbers; n=0,...,L-1}; N value is usually 1024. Wherein the acquisition signal f s (n) Divide into m groups of data with a length of N ea...
PUM
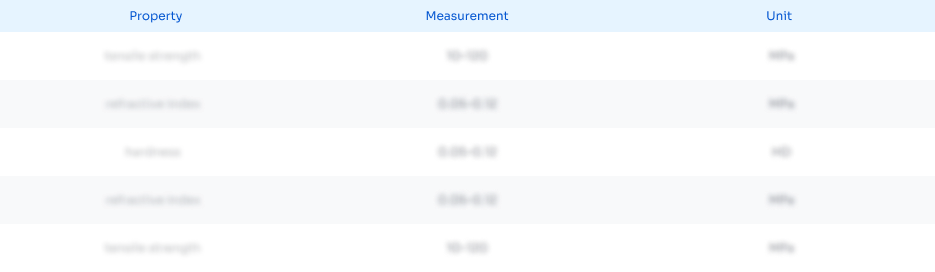
Abstract
Description
Claims
Application Information
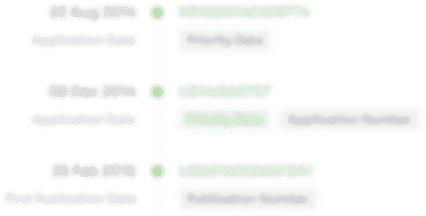
- R&D
- Intellectual Property
- Life Sciences
- Materials
- Tech Scout
- Unparalleled Data Quality
- Higher Quality Content
- 60% Fewer Hallucinations
Browse by: Latest US Patents, China's latest patents, Technical Efficacy Thesaurus, Application Domain, Technology Topic, Popular Technical Reports.
© 2025 PatSnap. All rights reserved.Legal|Privacy policy|Modern Slavery Act Transparency Statement|Sitemap|About US| Contact US: help@patsnap.com