Multi-linear large space feature extraction method
A feature extraction, large-spacing technology, applied in the multi-linear large-spacing feature extraction field, can solve the problems of destroying structural information, dimensional disaster, etc.
- Summary
- Abstract
- Description
- Claims
- Application Information
AI Technical Summary
Problems solved by technology
Method used
Image
Examples
Embodiment Construction
[0068] The present invention will be further described below in conjunction with the accompanying drawings and embodiments.
[0069] A multi-linear large-spacing feature extraction method, the specific operation steps are as follows:
[0070] (1) Preprocessing stage: Use the MPCA method to reduce the dimensionality of the entire tensor training set, and the obtained features are denoted as
[0071]
[0072]
[0073] in, It is the tensor after finding the direction where the original tensor data "changes" the most, is the found set of projection matrices, where Q n (n=1,...,N) indicates the dimensionality of the "n-mode" of the tensor after dimensionality reduction by MPCA; T is the transpose;
[0074] (2) Initialization stage of multi-linear projection matrix with large spacing: use the initialization method of full projection to find J * The eigendecomposition of , get its eigenvalues in descending order, initialize the projection matrix by J * ex-P n The ei...
PUM
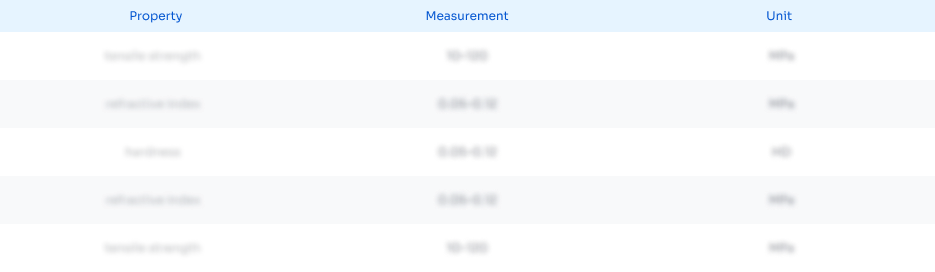
Abstract
Description
Claims
Application Information
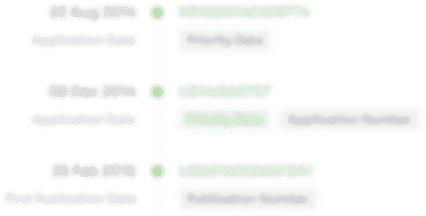
- R&D Engineer
- R&D Manager
- IP Professional
- Industry Leading Data Capabilities
- Powerful AI technology
- Patent DNA Extraction
Browse by: Latest US Patents, China's latest patents, Technical Efficacy Thesaurus, Application Domain, Technology Topic, Popular Technical Reports.
© 2024 PatSnap. All rights reserved.Legal|Privacy policy|Modern Slavery Act Transparency Statement|Sitemap|About US| Contact US: help@patsnap.com