Clustering method for high dimensional data based on Bayes mixed common factor analyzer
A technology of high-dimensional data and common factors, applied in the fields of electrical digital data processing, special data processing applications, instruments, etc.
- Summary
- Abstract
- Description
- Claims
- Application Information
AI Technical Summary
Problems solved by technology
Method used
Image
Examples
Embodiment Construction
[0095] In order to better illustrate the high-dimensional data clustering method based on the Bayesian Mixed Common Factor Analyzer (BMCFA) involved in the present invention, it is applied to the clustering of high-dimensional gene expression data in the field of bioinformatics. The data source to be clustered comes from the preprocessed 248 tissue samples provided by Yeoh et al., and the dimension of each sample is 50 (E. J. Yeoh et al. Classification, subtype discovery, and prediction of outcome in pediatric acute lymphoblastic leukemia by gene expression profiling, Cancer Cell, vol.1, no.2, pp.133-143, 2002.), namely N = 248, p = 50, .
[0096] There are 6 classes in this application, the class names and the number of samples in this class are: MLL (20 samples), T-ALL (43 samples), Hyperdip (64 samples), TEL-AML1 (79 samples ), E2A-PBX1 (27 samples), BCR-ABL (15 samples). Assume that the number of clusters and specific conditions are not known before clustering, and ...
PUM
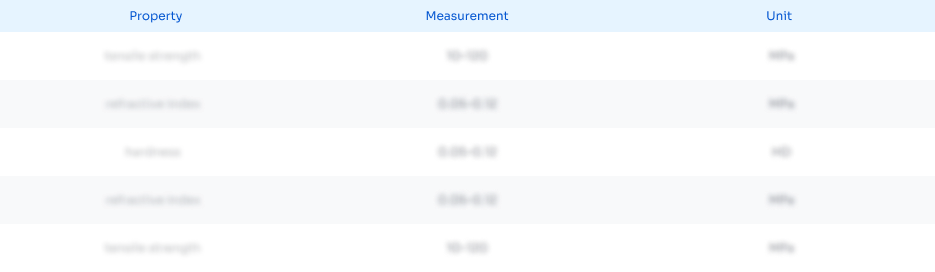
Abstract
Description
Claims
Application Information
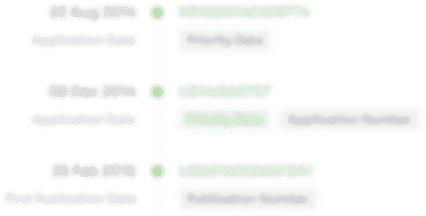
- R&D Engineer
- R&D Manager
- IP Professional
- Industry Leading Data Capabilities
- Powerful AI technology
- Patent DNA Extraction
Browse by: Latest US Patents, China's latest patents, Technical Efficacy Thesaurus, Application Domain, Technology Topic, Popular Technical Reports.
© 2024 PatSnap. All rights reserved.Legal|Privacy policy|Modern Slavery Act Transparency Statement|Sitemap|About US| Contact US: help@patsnap.com