A Weighted Image Compressive Sensing Method Based on General Hidden Markov Tree Model
A Hidden Markov Tree and Image Compression technology, applied in image enhancement, image data processing, instruments, etc., can solve the problems of high computational complexity of HMT model parameter estimation, reduce computational complexity, etc., to reduce computational complexity, The effect of reducing the possibility of repelling the model and improving the accuracy
- Summary
- Abstract
- Description
- Claims
- Application Information
AI Technical Summary
Problems solved by technology
Method used
Image
Examples
Embodiment Construction
[0069] The technique of the present invention is applicable to compressed sensing reconstruction of natural images. The uHMT model is introduced into the iterative weighted L1 method, the weight calculation method is improved, the shortcomings of the existing technology are overcome, and the original image is reconstructed. like figure 2 As shown, the present invention proposes a weighted image compression sensing method based on a general hidden Markov tree model, which comprises the following steps:
[0070] Step 1. Input the image A, and perform wavelet transformation on it to obtain the wavelet coefficient matrix X of A;
[0071] Step 2. Measure X through a Gaussian random matrix to obtain a measured value matrix Y;
[0072] Step 3. Divide the measurement matrix Y into blocks by column, each block is a one-dimensional column vector, record the kth column as Y k , each column is processed as follows;
[0073] Step 4. Set the number of iterations as i, and solve the P1 ...
PUM
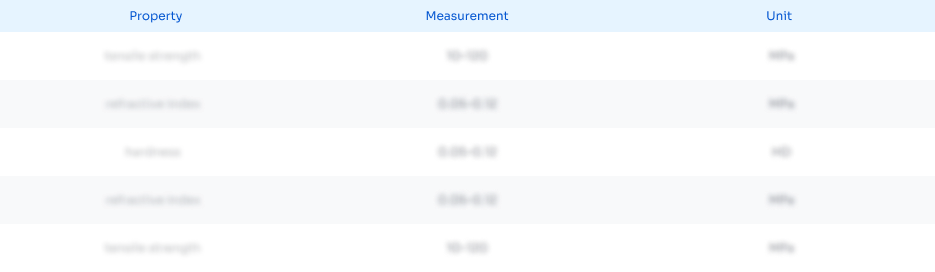
Abstract
Description
Claims
Application Information
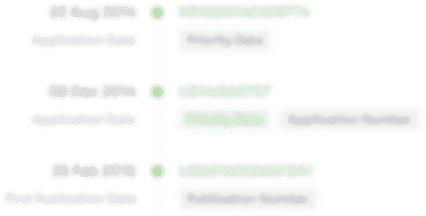
- R&D Engineer
- R&D Manager
- IP Professional
- Industry Leading Data Capabilities
- Powerful AI technology
- Patent DNA Extraction
Browse by: Latest US Patents, China's latest patents, Technical Efficacy Thesaurus, Application Domain, Technology Topic, Popular Technical Reports.
© 2024 PatSnap. All rights reserved.Legal|Privacy policy|Modern Slavery Act Transparency Statement|Sitemap|About US| Contact US: help@patsnap.com