Dual-model image decision fusion tracking method based on mutual updating of models
A decision-level fusion and mutual update technology, applied in image enhancement, image data processing, character and pattern recognition, etc., can solve the problems of poor imaging quality, lack of texture features, susceptibility to illumination changes, shadows, etc., to improve The effect of robustness
- Summary
- Abstract
- Description
- Claims
- Application Information
AI Technical Summary
Problems solved by technology
Method used
Image
Examples
Embodiment Construction
[0048] The present invention will be further described below in conjunction with accompanying drawing.
[0049] The experiment uses infrared and visible light images as OTCBVS sequence (infrared and visible light images have been registered), the image size is 320×240 pixels, the horizontal and vertical resolution is 96DPI, and the bit depth is 24.
[0050] The specific implementation steps are as follows:
[0051] Step (1). Extract the features of the initial frame infrared image and the initial frame visible light image
[0052] 1.1 Feature extraction of the initial frame infrared image:
[0053]Two features, grayscale color and gradient direction histogram (histogram of oriented gradients, HOG), are extracted from the initial frame infrared image. Gradient orientation histogram feature is a kind of local area descriptor, which can compose the target feature by calculating the gradient orientation histogram on the local area, which can well describe the edge of the target....
PUM
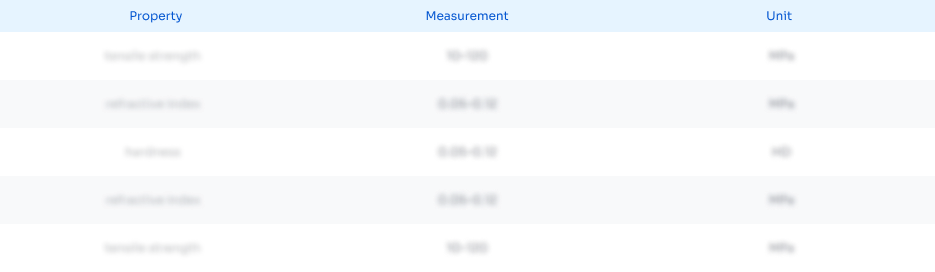
Abstract
Description
Claims
Application Information
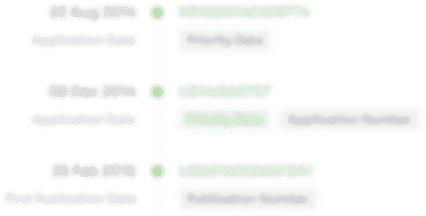
- R&D Engineer
- R&D Manager
- IP Professional
- Industry Leading Data Capabilities
- Powerful AI technology
- Patent DNA Extraction
Browse by: Latest US Patents, China's latest patents, Technical Efficacy Thesaurus, Application Domain, Technology Topic, Popular Technical Reports.
© 2024 PatSnap. All rights reserved.Legal|Privacy policy|Modern Slavery Act Transparency Statement|Sitemap|About US| Contact US: help@patsnap.com