Hierarchical clustering method and system based on multistage layered sampling
A hierarchical clustering, multi-stage technology, applied in relational databases, special data processing applications, instruments, etc., can solve the problems of low clustering accuracy and poor sample representativeness, and achieve high uncertainty, high representativeness, and improved The effect of accuracy
- Summary
- Abstract
- Description
- Claims
- Application Information
AI Technical Summary
Problems solved by technology
Method used
Image
Examples
Embodiment 1
[0053] Embodiment 1 of the present invention discloses a hierarchical clustering method based on multi-stage hierarchical sampling, such as figure 1 As shown, the method includes:
[0054] S1: Based on the preset input attribute set, a preset number of samples is randomly sampled from the data source, and the set formed by the collected preset number of samples is marked as the initial sample set.
[0055] Wherein, the data source may be background data that cannot be obtained directly but needs to be obtained by submitting a query through a query interface. In this embodiment, the data source is specifically a Deep Web data source.
[0056] This step S1 randomly collects a preset number of samples from the Deep Web. Generally, the number of samples randomly sampled at this stage is half of the total number of samples required for clustering. In this embodiment, assuming that a total of 2X samples need to be collected for clustering the target Deep Web (data source), X sample...
Embodiment 2
[0110] Embodiment 2 of the present invention discloses another flow of the hierarchical clustering method based on multi-stage hierarchical sampling, please refer to Figure 4 , on the basis of the method in Embodiment 1, it also includes:
[0111] S7: Set the iteration parameter x, and assign 1 to x.
[0112] This step S7 is specifically between steps S1 and S2.
[0113] S8: Judging whether the value of x is less than a preset number of iterations β. If the judgment result is yes, execute step S9; otherwise, if the judgment result is no, execute step S6.
[0114] S9: Add 1 to the value of x, combine the initial sample set, the representative sample set, and the uncertain sample set, and replace the initial sample set with the combined set as a new initial sample set. Go to step S2.
[0115] In order to collect samples with more information content from Deep Web, this embodiment continues to optimize the method of Embodiment 1. In the multi-stage sampling stage of stratifi...
Embodiment 3
[0117]Embodiment 3 continues to optimize the methods in Embodiment 1 and Embodiment 2. On the basis of the above embodiments, it further includes: suppressing excessive stratification of the strategy tree level during the process of constructing the strategy tree.
[0118] Because stratification may involve the problem of over-stratification, and over-stratification will lead to the deterioration of the results of stratified sampling. Therefore, in this embodiment, it is meaningful to test whether the variance of the output attribute is reduced by statistical hypothesis testing to determine whether to continue to stratify the strategy tree when building the strategy tree. The current node LN of the tree is layered. If it is meaningless, the layering of the current node LN in the policy tree is terminated. Specifically, it is tested by the following idea: when the potential splitting attribute P i When there is no significant connection with the output attribute set, the distr...
PUM
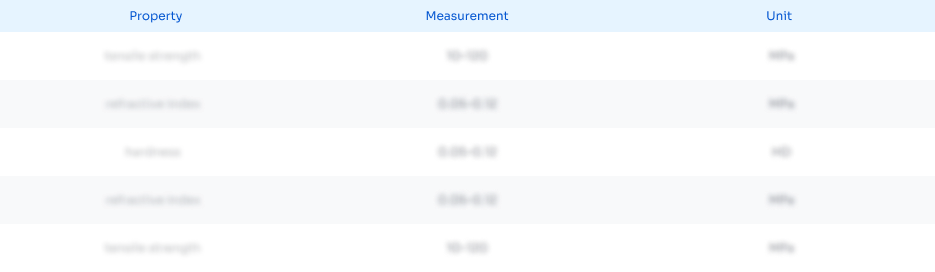
Abstract
Description
Claims
Application Information
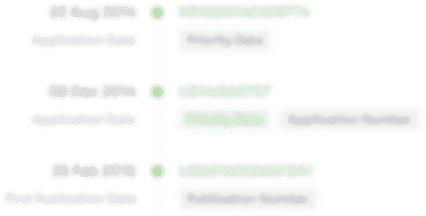
- R&D
- Intellectual Property
- Life Sciences
- Materials
- Tech Scout
- Unparalleled Data Quality
- Higher Quality Content
- 60% Fewer Hallucinations
Browse by: Latest US Patents, China's latest patents, Technical Efficacy Thesaurus, Application Domain, Technology Topic, Popular Technical Reports.
© 2025 PatSnap. All rights reserved.Legal|Privacy policy|Modern Slavery Act Transparency Statement|Sitemap|About US| Contact US: help@patsnap.com