Section traffic neural network prediction method based on EMD
A technology of neural network and prediction method, which is applied to biological neural network models, predictions, instruments, etc., can solve the problems of large amount of calculation and storage, and achieve the effects of saving time, improving prediction efficiency, and reducing calculation time
- Summary
- Abstract
- Description
- Claims
- Application Information
AI Technical Summary
Problems solved by technology
Method used
Image
Examples
Embodiment
[0137] This paper analyzes and selects the passenger flow data of the section from Xidan to Fuxingmen of Beijing Metro Line 1 (downlink) measured on weekdays. Such as Figure 4 as shown, Figure 4 It is (example) 2012.3.5-3.9 Beijing Subway Line 1 (downlink) Xidan-Fuxingmen cross-section passenger flow statistics map for every 30 minutes. The present invention predicts the passenger flow at the Xidan-Fuxingmen section on March 9, 2012 based on the passenger flow data at the Xidan-Fuxingmen section from 2012.3.5 to 2012.3.8.
[0138] An EMD-based cross-sectional passenger flow neural network prediction method, the method steps are as follows:
[0139] Step 1: Obtain data eigenmode function components
[0140] Decompose the EMD empirical mode on the above data, as follows Figure 5 as shown, Figure 5 is a schematic diagram of the (embodiment) EMD empirical decomposition model. From Figure 5 It can be seen from the figure that the original cross-sectional passenger flow ...
PUM
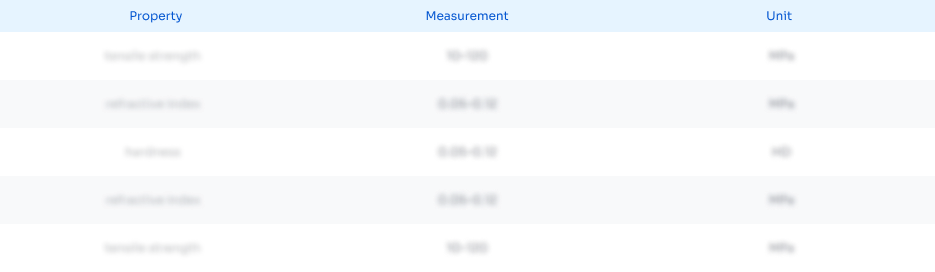
Abstract
Description
Claims
Application Information
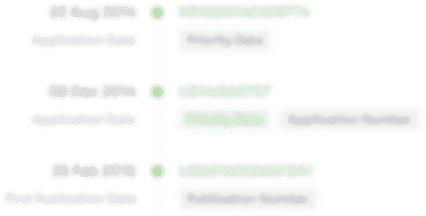
- R&D Engineer
- R&D Manager
- IP Professional
- Industry Leading Data Capabilities
- Powerful AI technology
- Patent DNA Extraction
Browse by: Latest US Patents, China's latest patents, Technical Efficacy Thesaurus, Application Domain, Technology Topic.
© 2024 PatSnap. All rights reserved.Legal|Privacy policy|Modern Slavery Act Transparency Statement|Sitemap